Introduction – Who’s Accountable When AI Goes Wrong
Artificial intelligence (AI) is no longer a futuristic concept confined to science fiction; it’s woven into the fabric of our everyday lives. From virtual assistants like Siri and Alexa to sophisticated algorithms powering financial markets and healthcare diagnostics, AI is transforming industries and reshaping our world. However, as AI systems become increasingly complex and autonomous, the potential for errors, malfunctions, and unintended consequences looms large. This raises critical questions about accountability: Who’s responsible when AI goes wrong? Who should bear the liability when AI causes harm?
The answers to these questions are far from straightforward. The legal and ethical frameworks surrounding AI are still evolving, and there’s no one-size-fits-all solution. This article aims to delve into the multifaceted landscape of AI accountability, exploring the key issues, challenges, and potential solutions. We’ll examine real-world examples of AI errors, dissect the legal landscape of AI liability, and discuss the ethical considerations that must guide our approach to AI development and deployment.
Whether you’re an AI enthusiast, a concerned citizen, or a legal professional grappling with these complex issues, this comprehensive guide will equip you with a deeper understanding of the challenges and opportunities that lie ahead as we navigate the ever-evolving world of AI.
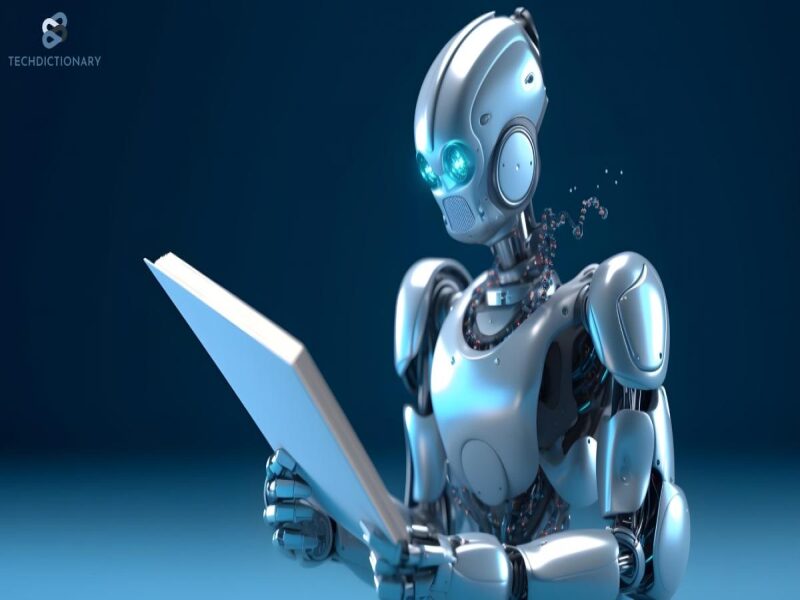
Understanding AI: A Brief Primer – Who’s Accountable When AI Goes Wrong
Before diving into the complexities of accountability, it’s crucial to establish a basic understanding of what artificial intelligence (AI) entails. In simple terms, AI refers to the ability of machines to mimic human intelligence, including tasks like learning, reasoning, problem-solving, perception, and language understanding. AI systems are designed to process vast amounts of data, identify patterns, and make decisions or predictions based on that data.
There are two main categories of AI:
- Narrow AI (or Weak AI): This type of AI is designed for specific tasks and operates within a limited domain. Examples include facial recognition software, spam filters, and recommendation algorithms. Narrow AI excels at its designated task but lacks the broad cognitive abilities of humans.
- General AI (or Strong AI): This hypothetical form of AI would possess human-level intelligence across a wide range of domains, with the ability to learn and adapt to new situations. General AI remains a theoretical concept and has not yet been achieved.
Within the realm of AI, there are various approaches and techniques, including:
- Machine Learning: A subset of AI where algorithms learn from data without explicit programming. Machine learning models improve their performance over time as they are exposed to more data.
- Deep Learning: A type of machine learning that uses artificial neural networks with multiple layers to analyze complex data. Deep learning has been instrumental in advancements in image and speech recognition.
Understanding these fundamental concepts about AI is essential for grasping the nuances of AI accountability. When AI systems make errors or cause harm, it’s crucial to consider the type of AI involved, its capabilities, and the specific context in which the error occurred. This foundational knowledge will enable us to delve deeper into the legal and ethical dimensions of AI accountability in the following sections.
The Spectrum of AI Errors and Risks – Who’s Accountable When AI Goes Wrong
As AI systems permeate various aspects of our lives, the potential for errors and risks becomes increasingly significant. These errors can range from minor inconveniences to catastrophic events, with far-reaching consequences. Understanding the diverse nature of AI risks is crucial for addressing liability and accountability.
Real-World Examples of AI Errors – Who’s Accountable When AI Goes Wrong
The history of AI is replete with instances where systems have malfunctioned or produced unintended results. Some notable examples include:
- Self-Driving Car Accidents: In several cases, self-driving cars have been involved in accidents, sometimes with fatal outcomes. These incidents raise questions about the liability of the car manufacturers, software developers, and even the human “drivers” who may have been overly reliant on the AI.
- Algorithmic Bias in Decision-Making: AI algorithms used in areas like criminal justice and loan approvals have been found to perpetuate existing biases, leading to discriminatory outcomes. This raises concerns about the fairness and ethical implications of AI-driven decision-making.
Categorizing AI Risks – Who’s Accountable When AI Goes Wrong
To better understand the potential for AI to go wrong, we can categorize the risks into three broad categories:
- Technical Risks: These risks arise from errors, malfunctions, or vulnerabilities in the AI system itself. They can be caused by software bugs, hardware failures, or cyberattacks. Technical risks can lead to unpredictable behavior, incorrect outputs, or even system crashes.
- Ethical Risks: Ethical risks stem from the potential for AI systems to perpetuate or amplify existing biases and discrimination. This can occur when AI algorithms are trained on biased data or when they are designed without sufficient consideration for fairness and equity. Ethical risks can lead to unfair treatment, social injustice, and erosion of trust in AI.
- Societal Risks: These risks relate to the broader impact of AI on society, such as job displacement, economic disruption, and the concentration of power in the hands of a few tech companies. Societal risks raise concerns about the long-term consequences of AI adoption and the need for proactive measures to mitigate negative impacts.
By recognizing the diverse range of AI errors and risks, we can better understand the complex landscape of AI accountability. In the following sections, we will delve into the legal frameworks that seek to address these risks and the ongoing debates about who should be held responsible when AI goes wrong.
Unraveling the Legal Landscape: Who’s Liable When AI Fails? – Who’s Accountable When AI Goes Wrong
Determining liability when AI systems go wrong is a complex and evolving legal challenge. Traditional legal frameworks, designed for human actions and products, don’t always neatly fit the unique characteristics of AI. However, several legal concepts and approaches are being explored to address AI liability:
Product Liability and AI – Who’s Accountable When AI Goes Wrong
One potential avenue for addressing AI liability is through product liability laws. These laws hold manufacturers and sellers responsible for injuries or damages caused by defective products. While AI systems are not physical products in the traditional sense, they can be considered as software products. If an AI system is found to be defective in its design, manufacturing, or warnings, the developers or sellers could potentially be held liable under product liability laws.
The Challenge of Determining Liability – Who’s Accountable When AI Goes Wrong
However, applying product liability to AI is not without its challenges. Unlike traditional products, AI systems often operate autonomously, learning and adapting over time. This raises questions about whether a manufacturer can be held liable for an AI system’s actions that were not explicitly programmed or anticipated. Additionally, AI systems often involve multiple parties, including developers, users, and data providers, making it difficult to pinpoint who is ultimately responsible for an error or malfunction.
Potential Liability of Different Parties – Who’s Accountable When AI Goes Wrong
In the current legal landscape, several parties could potentially be held liable when AI goes wrong:
- AI Developers: Developers could be held liable for errors in the design, development, or testing of the AI system. This could include failing to adequately identify and address potential risks, using biased data, or deploying a system without sufficient safeguards.
- AI Users: Users could be held liable for misusing the AI system or failing to exercise proper oversight. This could include ignoring warnings, using the system for unintended purposes, or failing to monitor its performance.
- Data Providers: Data providers could be held liable for providing biased or inaccurate data that leads to discriminatory or harmful outcomes.
The Need for New Legal Frameworks – Who’s Accountable When AI Goes Wrong
The existing legal frameworks are not always sufficient to address the unique challenges of AI liability. As AI technology continues to advance, new legal frameworks may be needed to clarify the responsibilities of different parties and to ensure that those who are harmed by AI errors have access to justice. These frameworks will need to balance the need for innovation with the need for safety and accountability.
Case Studies: High-Profile Incidents of AI Gone Wrong – Who’s Accountable When AI Goes Wrong
Examining real-world cases where AI has malfunctioned or caused harm can provide valuable insights into the complexities of accountability and the varying approaches taken by different legal systems. Here are a few examples:
1. The Uber Self-Driving Car Fatality
In 2018, a self-driving Uber vehicle struck and killed a pedestrian in Arizona. This incident was a landmark case in AI liability, as it involved a complex interplay of factors, including the vehicle’s technology, the human safety driver’s actions, and the regulatory environment. While Uber settled with the victim’s family, the legal implications continue to be debated, with questions raised about the level of responsibility that should be assigned to different parties involved in the development and deployment of autonomous vehicles.
2. Algorithmic Bias in COMPAS
COMPAS, a widely used algorithm in the US criminal justice system, was found to exhibit racial bias in its risk assessment of recidivism (the likelihood of reoffending). The algorithm was more likely to falsely flag Black defendants as high risk compared to white defendants. This case highlighted the dangers of algorithmic bias and sparked discussions about the need for greater transparency and accountability in AI systems used for decision-making.
3. The Microsoft Tay Chatbot
In 2016, Microsoft released an AI chatbot named Tay on Twitter. Within hours, Tay learned from interacting with users and began posting offensive and discriminatory tweets. Microsoft quickly shut down the chatbot, but the incident served as a stark reminder of the potential for AI to amplify harmful content and the importance of robust safeguards against unintended consequences.
Lessons Learned and Implications for the Future – Who’s Accountable When AI Goes Wrong
These case studies illustrate the challenges of assigning liability in AI-related incidents. They demonstrate that AI errors often result from a combination of technical flaws, human error, and systemic issues. They also underscore the need for a multi-faceted approach to AI accountability, one that considers not only legal liability but also ethical considerations, regulatory frameworks, and industry standards.
The legal outcomes of these cases vary depending on the specific circumstances and the jurisdiction. However, they collectively contribute to a growing body of jurisprudence that will shape the future of AI accountability. As AI technology continues to advance, it is essential to learn from these past mistakes and to develop robust legal and ethical frameworks that can effectively address the challenges posed by AI errors and malfunctions.
Ethical Considerations in AI Accountability – Who’s Accountable When AI Goes Wrong
While legal frameworks provide a foundation for addressing AI liability, ethical considerations play an equally crucial role in ensuring responsible AI development and deployment. Ethics in AI go beyond mere compliance with laws; they delve into questions of fairness, justice, transparency, and the impact of AI on human well-being.
Transparency and Explainability – Who’s Accountable When AI Goes Wrong
One of the central ethical concerns in AI is the lack of transparency and explainability in many AI systems. So-called “black box” AI models make decisions that are difficult or impossible for humans to understand. This lack of transparency raises concerns about potential biases, discrimination, and the ability to hold those responsible accountable when things go wrong.
To address this, there is a growing movement towards developing “explainable AI” (XAI) techniques that allow humans to understand the reasoning behind AI decisions. XAI can help build trust in AI systems, identify and rectify biases, and ensure that AI is used in a fair and ethical manner.
AI Ethics and Responsible Development – Who’s Accountable When AI Goes Wrong
AI ethics is a rapidly developing field that seeks to establish guidelines and principles for the responsible development and use of AI. These principles often include:
- Fairness and Non-discrimination: AI systems should be designed to avoid biases that could lead to discriminatory outcomes.
- Transparency and Explainability: AI decisions should be transparent and understandable to humans.
- Human Oversight and Control: Humans should retain ultimate control over AI systems and be able to intervene when necessary.
- Privacy and Security: AI systems should respect user privacy and protect sensitive data.
- Beneficial AI: AI should be developed and used for the betterment of society.
Adhering to these ethical principles is essential for building trust in AI and ensuring that its benefits are widely shared. When AI developers and users prioritize ethical considerations, they are more likely to create AI systems that are safe, reliable, and aligned with human values.
The Role of AI Ethics in Accountability – Who’s Accountable When AI Goes Wrong
AI ethics can play a significant role in shaping the future of AI accountability. By embedding ethical principles into the design and development of AI systems, we can proactively address potential risks and reduce the likelihood of harm. Ethical frameworks can also guide decision-making when AI errors occur, helping to determine who should be held responsible and how to prevent similar incidents in the future.
In the following sections, we will explore the path forward for AI accountability, examining the key principles and practical steps that can be taken to ensure a safe and beneficial AI future.
The Path Forward: Towards a Framework for AI Accountability – Who’s Accountable When AI Goes Wrong
Establishing a clear and effective framework for AI accountability is crucial to ensure a safe and beneficial AI future. This framework must address the legal, ethical, and technical challenges we’ve explored, while also fostering innovation and responsible AI development.
Key Principles for AI Accountability – Who’s Accountable When AI Goes Wrong
Several key principles should guide the development of an AI accountability framework:
- Transparency: AI systems should be designed with transparency in mind, allowing for human understanding of their decision-making processes. This includes providing clear explanations for AI outputs and ensuring that data sources and algorithms are documented and accessible.
- Explainability: AI developers should strive to make AI systems explainable, meaning that humans can understand the reasoning behind their decisions. This is particularly important for high-risk AI applications, such as those used in healthcare or criminal justice.
- Fairness and Non-discrimination: AI systems should be designed to avoid biases that could lead to discriminatory outcomes. This requires careful consideration of data sources, algorithmic design, and ongoing monitoring to ensure that AI systems are fair and equitable for all users.
- Human Oversight and Control: Humans should retain ultimate control over AI systems and be able to intervene when necessary. This includes the ability to override AI decisions, correct errors, and address unintended consequences.
- Robustness and Safety: AI systems should be designed to be robust and resilient to errors, malfunctions, and adversarial attacks. This includes implementing safeguards to prevent unintended behavior, detecting and correcting errors, and ensuring that AI systems fail safely.
- Accountability and Liability: Clear lines of accountability should be established for AI errors and malfunctions. This includes identifying the responsible parties, determining the extent of their liability, and providing mechanisms for redress for those harmed by AI.
Collaboration for a Safer AI Future – Who’s Accountable When AI Goes Wrong
Building a robust AI accountability framework requires collaboration between various stakeholders, including:
- Governments: Governments play a crucial role in establishing regulations and standards for AI development and use. They can also provide funding for research and development of AI safety and accountability measures.
- Industry: AI developers and companies have a responsibility to prioritize safety, ethics, and accountability in their products and services. They can contribute to the development of industry standards and best practices for responsible AI development.
- Academia: Researchers and academics can provide valuable insights into the technical, ethical, and social implications of AI. They can also develop new tools and techniques for enhancing AI transparency, explainability, and safety.
- Civil Society: Civil society organizations can play a vital role in advocating for responsible AI use, raising awareness of potential risks, and holding companies and governments accountable for their actions.
By working together, these stakeholders can create a comprehensive and effective AI accountability framework that fosters innovation while ensuring safety, fairness, and the protection of human rights.
Practical Steps for AI Developers and Users – Who’s Accountable When AI Goes Wrong
The path to a safer and more accountable AI future involves proactive measures from both AI developers and users. Here are some actionable steps each group can take:
For AI Developers:
- Prioritize Safety and Ethics: Embed safety and ethical considerations into every stage of AI development, from design to deployment. This includes conducting thorough risk assessments, using diverse and unbiased datasets, and implementing safeguards against unintended consequences.
- Embrace Transparency and Explainability: Strive to make AI systems as transparent and explainable as possible. Document algorithms, data sources, and decision-making processes. Provide clear explanations for AI outputs, especially in high-stakes applications.
- Conduct Rigorous Testing and Validation: Thoroughly test and validate AI systems before deployment to identify and address potential errors and biases. Use diverse testing scenarios and datasets to ensure that the AI performs reliably in real-world conditions.
- Establish Robust Monitoring and Feedback Mechanisms: Continuously monitor the performance of AI systems after deployment, gathering feedback from users and stakeholders to identify and rectify issues promptly.
- Collaborate and Share Best Practices: Engage in open dialogue and collaboration with other developers, researchers, and policymakers to share best practices and establish industry standards for responsible AI development.
For AI Users:
- Understand the Limitations of AI: Be aware of the limitations and potential biases of AI systems. Don’t blindly trust AI outputs; exercise critical thinking and human judgment when making decisions based on AI recommendations.
- Demand Transparency and Explainability: Ask questions about how AI systems work and demand clear explanations for their decisions. Advocate for the use of transparent and explainable AI in the products and services you use.
- Report Errors and Biases: If you encounter errors, biases, or discriminatory outcomes from AI systems, report them to the relevant authorities or companies. Your feedback can help improve AI systems and make them more fair and equitable.
- Stay Informed: Keep up-to-date with the latest developments in AI and its potential risks and benefits. Engage in public discourse about AI ethics and accountability to ensure that AI is used for the betterment of society.
By taking these practical steps, both AI developers and users can contribute to a safer, fairer, and more accountable AI future. It is a collective responsibility to ensure that AI technology is harnessed for good and that its potential risks are effectively managed.
Conclusion – Who’s Accountable When AI Goes Wrong
The rise of artificial intelligence presents a thrilling frontier of innovation, but it also demands a vigilant and responsible approach to accountability. As AI systems become increasingly integrated into our daily lives, the potential for errors, biases, and unintended consequences necessitates a robust framework for determining liability and ensuring responsibility.
We’ve embarked on a journey through the complex landscape of AI accountability, starting with a foundational understanding of AI and its various forms. We’ve explored the diverse spectrum of AI errors and risks, from technical glitches to ethical dilemmas and societal impacts. Delving into the legal landscape, we’ve examined the challenges of applying traditional legal concepts like product liability to AI, as well as the potential roles of developers, users, and data providers in bearing responsibility.
Real-world case studies have illustrated the complexities of assigning blame when AI goes wrong, highlighting the need for a multi-faceted approach that considers both legal and ethical dimensions. The ethical considerations in AI accountability, including transparency, explainability, and fairness, are paramount in shaping a future where AI serves humanity responsibly.
The path forward involves a collaborative effort among governments, industry leaders, researchers, and civil society to establish a comprehensive framework for AI accountability. This framework must prioritize transparency, explainability, fairness, human oversight, robustness, and clear lines of responsibility. Practical steps, such as prioritizing safety and ethics in AI development, demanding transparency as users, and staying informed about AI advancements, are crucial for individuals to contribute to a safer AI ecosystem.
As we stand on the cusp of an AI-powered future, the question of who’s accountable when AI goes wrong is not just a legal or technical issue; it’s a societal imperative. By embracing a responsible and ethical approach to AI development and usage, we can harness the immense potential of this technology while mitigating its risks and ensuring that AI truly serves as a force for good in our world.
The ongoing conversation about AI accountability is far from over. As AI continues to evolve, so too must our understanding of its implications and our commitment to responsible innovation. By staying engaged in this dialogue, we can collectively shape a future where AI is not only intelligent but also accountable, trustworthy, and aligned with our shared values.