2024 is a revolutionary year in artificial intelligence because various AI tools were unveiled this year. Even though just two months has been passed this year, a significant amount of unbreakable AI innovation has come to the world to revolutionize it. One of the unforgettable AI tools that was released this year is Gemini 5. It is a multimodal large language model that Google and DeepMind developed. OpanAI introduced another incredible AI tool on February 15, 2024. The name is SORA. Once again, I astounded everyone with the reveal of Sora. According to OpenAI, this technology is capable of creating videos in up to a minute from a given text prompt.
On February 15, 2024, OpenAI, which had astonished the world by announcing ChatGPT in late 2022. Once again, they stunned the world with the unveiling of Sora. This technology, capable of creating videos up to a minute long from a text prompt, is undeniably set to be a breakthrough.
In this article, we are trying to give you a full understanding of these tools. And also what their capabilities are, and what kinds of things can be generated from these tools, methodologies, and research that are undertaken behind this astonishing technology, based on the technical report released by OpenAI.
The simple meaning of the word “Sora” is “sky”. It is a Japanese word. Also, this AI tool has not been officially released. But OpenAI is sharing their research progress early to start working with and getting feedback from people outside of them and to give the public a sense of what AI capabilities are on the horizon. We are preparing this article according to the OpenAI newsletter. And we will update this article just in time.
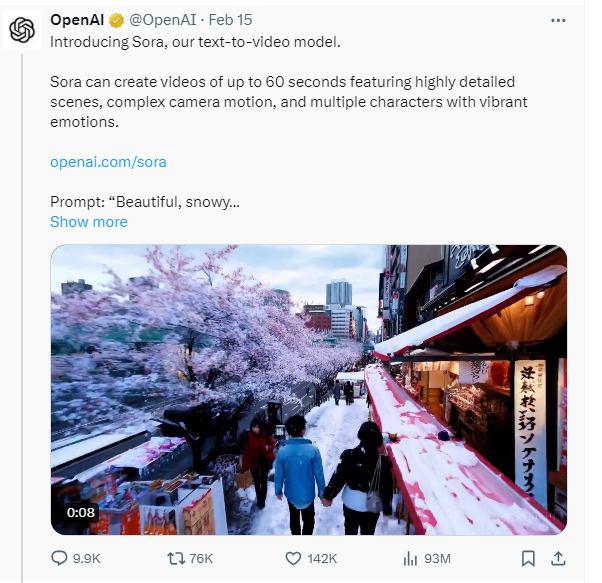
What is Sora?
Sora is an advanced AI model that has the capability of text-to-video conversion. It is not just a video conversion model; it has an incredible range of capabilities and applications. It will illustrate a new horizon in model AI technology. It can create high-realistic videos up to one minute long by maintaining excellent visual quality and accurately reproducing user instructions.
Sora can create complex senses from Real-world Data
Sora can understand how elements are described in the prompts and how they exist in the real world. This enables the model to faithfully capture the gestures and actions that users plan to perform within films. For example, imagine that you wanted to generate video shots to show a person working on an urban street. And the camera is moving in front of that person with him, and a large number of people are working here and there behind the main character. Then you can generate such a video by giving a text prompt.
This model accurately represents user-intended movements and actions within the videos, and it has an incredible ability to give high-quality output that exactly matches the correct object movement and the scall of the object in natural phenomena. It also faithfully captures the fine details of various people, different movement styles, and the particulars of themes and backgrounds.
Prompt: A stylish woman walks down a Tokyo street filled with warm glowing neon and animated city signage. She wears a black leather jacket, a long red dress, and black boots, and carries a black purse. She wears sunglasses and red lipstick. She walks confidently and casually. The street is damp and reflective, creating a mirror effect of the colorful lights. Many pedestrians walk about.
Before Sora, creating videos with Genaraive AI presented some challenging obstacles in terms of preserving the repeatability and consistency of many senses. Because it was difficult for the prior AI model to generate each scene or frame separately and correctly inherit them to the next scene, it was unable to fully understand past contexts and details. However, this AI model can maintain a narrative and accurate sequence. Because it’s a deep understanding of language with visual context and given text prompts. Also, it can depict characters in the video as expressive, capturing their personalities and feelings based on the prompts provided.
Prompt: Drone view of waves crashing against the rugged cliffs along Big Sur’s garay point beach. The crashing blue waters create white-tipped waves, while the golden light of the setting sun illuminates the rocky shore. A small island with a lighthouse sits in the distance, and green shrubbery covers the cliff’s edge. The steep drop from the road down to the beach is a dramatic feat, with the cliff’s edges jutting out over the sea. This is a view that captures the raw beauty of the coast and the rugged landscape of the Pacific Coast High.
Technology Behind the SORA
This AI model has been developed upon a foundation of previous studies in image generation modeling. Previously, several studies have been conducted to test various methods, such as recurrent networks, generative adversarial networks (GANs), autoregressive transformers, and diffusion models. However, these have often focused on a narrow category of visual data shooter videos or videos of a fixed size. But Sora has broken those limitations. Beyond these restrictions, Sora has undergone substantial development to produce films with a wide range of lengths, aspect ratios, and resolutions. In this section, we will see what the most important technologies are to bring these advancements to Sora. There are four technologies we can identify for this. Those are,
01 Transformer
Vaswani et al. (2017), “Attention is all you need.”
One kind of neural network architecture called a transformer converts an input sequence into an output sequence. They achieve this by observing the connections between the sequence’s constituent parts and gaining context. Take into consideration the following input sequence, for instance: “What is the color of the sea?” The relevance and connection between the terms color, sea, and blue are determined by the transformer model through the usage of an internal mathematical representation. The output, “The sky is blue,” is produced using that knowledge. This technology has revolutionized the field of natural language processing (NLP). Vaswani et al. made the initial proposal for it in 2017. The difficulties that conventional Recurrent Neural Networks (RNNs) and Convolutional Neural Networks (CNNs) encountered were greatly overcome by this model, which now serves as an inventive approach to support numerous ground-breaking technologies.
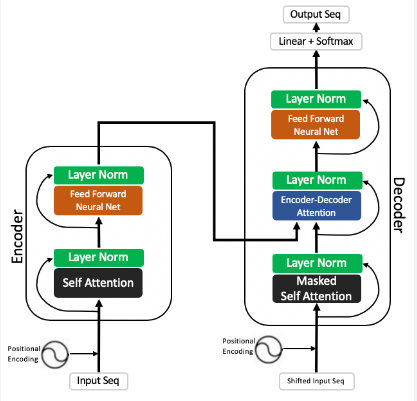
Image Source: From the article written by Pranay Dugar in Medium
Issues with RNNs:
01. The problem of long-term dependencies: RNNs have difficulty capturing dependencies over extended periods, despite their theoretical ability to communicate information over time.
02. Limitations on Parallelization: Sequential processing, or processing words or phrases in a text one by one and in order, is required since each step of an RNN depends on the result of the preceding phase. This means that the benefits of parallel processing provided by contemporary computer architectures cannot be used. As a result, training with large datasets becomes ineffective.
Issues with CNNs:
01. Fixed receptive field size: Although CNNs are excellent at extracting local features, they are not as good at capturing long-distance dependencies across the context because of their limited receptive field size.
02. Modeling the hierarchical structure of natural language is difficult: Direct modeling of a language’s hierarchical structure is difficult and may not provide thorough enough contextual knowledge.
New features of this Technology
01. Attention Mechanism: This mechanism allows for the direct capture of complex dependencies and large amounts of context by enabling the direct modeling of dependencies between any points in the sequence.
02. Realization of parallelization: This model can achieve a high degree of computation parallelization because the input data is processed all at once, greatly speeding up training on big datasets.
03. Variable receptive field: When a task or set of data calls for it, the attention mechanism enables the model to dynamically adapt the size of the “receptive field,” so that it can naturally concentrate on local information in some situations and take into account broad context in others.
2. Vision Transformer (ViT)
Dosovitskiy et al. (2020) explored the application of Transformer principles, renowned for revolutionizing natural language processing (NLP), to image recognition. This study has opened new horizons by revolutionizing the artificial intelligence sector. Also, this study has found that an image is worth 16*16 words; this word can be used for image recognition by transformers.
What are the Token and Patch in ViT?
Tokens in the Transformer paper of the original design mostly represent segments of words or phrases, and a thorough comprehension of the content of the sentence can be obtained by examining the connections between these tokens. Images are split into 16×16 patches for the sake of this study’s application of the token concept to visual data. The Transformer treats each patch as a “token.” By using this method, the model can perceive and comprehend the full image by learning how each patch is related to the rest of the image. With its ability to capture spatial relationships in any image, it overcomes the constraints of the limited receptive field size of classic CNN models employed in image
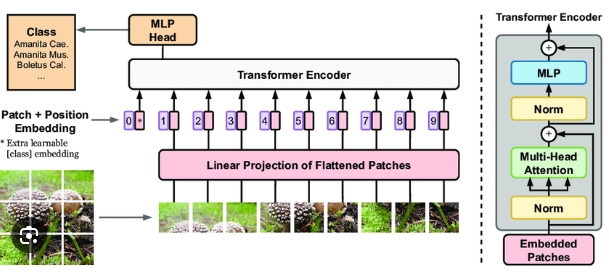
Image Source:- https://www.researchgate.net/figure/Vision-Transformer-architecture-main-blocks-First-image-is-split-into-fixed-size_fig1_357885173
3. Video Vision Transformer (ViViT)
Arnab, et al. (2021), “Vivit: A video vision transformer.”
ViViT applies the Vision Transformer concept to multidimensional video data, expanding upon it even more. Due to the combination of dynamic information that changes over time (temporal components) and static image information (spatial elements), video data is more complex. Videos are broken down into spatiotemporal patches by ViViT, which then uses these patches as tokens in the Transformer model. ViViT can now record both static and dynamic aspects of a video concurrently and simulate the intricate interactions between them thanks to the addition of spatiotemporal patches.
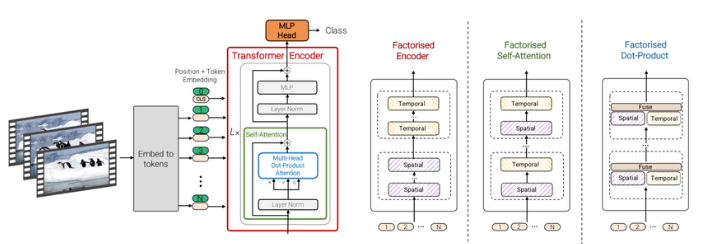
Image source:- https://www.researchgate.net/figure/Video-Vision-Transformer-architecture-In-this-study-the-ViViT-transformer-is-implemented_fig4_373379584
4. Masked Autoencoders (MAE)
The Masked Autoencoder, a self-supervised pre-training technique, was used in this work to significantly decrease the normally high computing costs and inefficiencies in training on massive data sets linked to high dimensionality and tremendous amounts of data.
In particular, the network is trained to predict the information of the hidden sections by masking portions of the input image. This process makes it easier for the network to learn critical elements and structures within the image and produces rich representations of visual data. This procedure has decreased processing costs, improved the adaptability of various visual data types and jobs, and improved the efficiency of data compression and representation
This study’s methodology has a significant relationship to BERT’s (Bidirectional Encoder Representations from Transformers) development of language models. While BERT used Masked Language Modeling (MLM) to enable a deep contextual understanding of text data, He et al. used a similar masking technique to get a richer comprehension and representation of images in visual data.
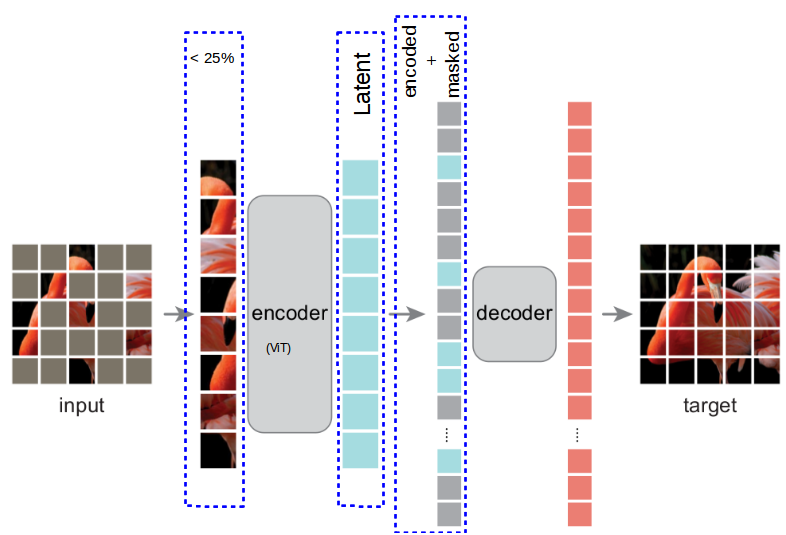
Image Source: https://mchromiak.github.io/articles/2021/Nov/14/Masked-Autoencoders-Are-Scalable-Vision-Learners/
5. Native Resolution Vision Transformer (NaViT)
This model was created to increase the range of images with any aspect ratio or resolution that may be used with Vision Transformer (ViT).
Until now, there has been no effective challenge to the widely used and flawed method of scaling images to a set resolution before using computer vision models to process them. However, flexible sequence-based modeling is provided by models like the Vision Transformer (ViT), allowing for different input sequence lengths.
NaViT (Native Resolution ViT) makes use of this benefit by processing inputs with arbitrary resolutions and aspect ratios through the use of sequence packing during training. Improved training efficiency for large-scale supervised and contrastive image-text pretraining is demonstrated, in addition to flexible model utilization. Improved results on robustness and fairness benchmarks are obtained by applying NaViT to standard tasks like object detection, semantic segmentation, and image and video classification in an efficient manner.
What are the Challenges of Traditional ViT
By applying the transformer concept to image identification tasks, the Vision Transformer developed a novel method by segmenting images into fixed-size patches and treating these patches as tokens. This method, however, relied on models that were optimized for particular aspect ratios or resolutions, which requires model readjustment for images of various sizes or forms. Given that real-world programs frequently need to handle images of various sizes and aspect ratios, this was a major limitation.
Features of NaViT
Images of any aspect ratio or resolution can be processed effectively by NaViT, enabling direct entry of the data into the model without the need for preprocessing. Sora uses this same adaptability to handle films and images of different sizes and forms, greatly increasing flexibility and adaptability.
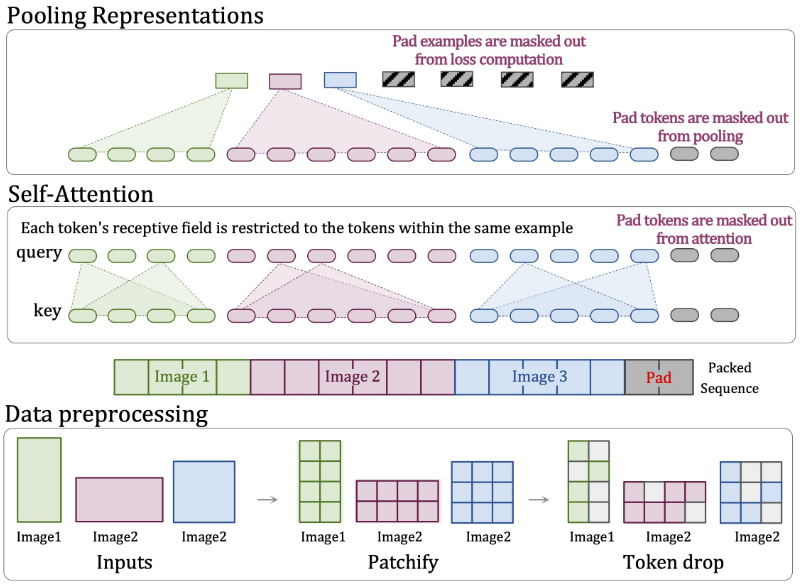
Image Source:-https://paperswithcode.com/paper/patch-n-pack-navit-a-vision-transformer-for
6. Diffusion Models
Diffusion models, along with the transformer, provide the core technology that powers Sora. The theoretical framework of diffusion models—a deep learning model based on non-equilibrium thermodynamics—was established by this research. The idea of a diffusion process that begins with random noise (input devoid of any pattern) and progressively eliminates it to produce data that resembles real images or videos was first presented by diffusion models.
Consider beginning with only a few random dots and seeing them progressively change into videos of impressive people or landscapes. Later, this method was used to generate complicated data, including sounds and visuals, which helped to create excellent generative models.
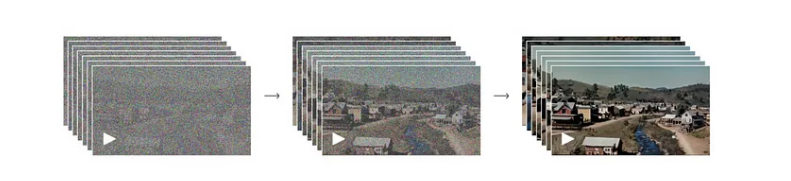
Image Source: OpenAI Technical Report of SORA
Denoising Diffusion Probabilistic Models (DDPM) is a class of useful data-generating models that were built by building upon the theoretical framework proposed by Sohl-Dickstein et al. (2015). Diffusion models are effective; this model has produced particularly noteworthy outcomes in high-quality image production.
How does a diffusion model work in SORA?
Training the machine learning model is not an easy task. Normally, it needs a lot of labeled data. As an example, labeled data can be represented as “This is an image of a lion.”. Then a machine learning model can learn from that data. However, diffusion models are also capable of learning from unlabeled data, which lets them make use of the large amount of visual content on the internet to produce different kinds of videos. To put it another way, Sora can watch a variety of images and videos and figure out, “This is what a typical video looks like.”
7. Latent Diffusion Models
This model makes a major addition to the field of diffusion model based high resolution image synthesis. It suggests a technique that, by using diffusion models in the latent space, maintains quality while drastically lowering computational costs when compared to direct high-resolution image production. Put another way, it shows that it is possible to accomplish this with less computational power by encoding and applying the diffusion process to data represented in the latent space—a lower-dimensional space containing compressed representations of images—rather than directly manipulating images.
Using this technique, Sora compresses and then decomposes video data into spatiotemporal patches. This lower-dimensional latent space is created from the temporal and spatial data of the films. A major factor in Sora’s capacity to produce visual content of excellent quality more quickly is its effective data processing and production capability in the latent space.
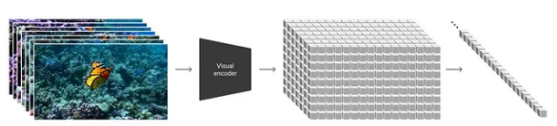
Image Source: OpenAI Technical Report of SORA
8. Diffusion Transformer (DiT)
Perhaps the most important research toward realizing Sora is this one. As stated in the OpenAI technical study, Sora uses a diffusion transformer (DiT) rather than a standard transformer. Perhaps the most important research toward realizing Sora is this one. As stated in the OpenAI technical report, Sora uses a diffusion transformer (DiT) rather than a standard transformer.
In the research, a Transformer structure was developed as a substitute for the U-net component, which is frequently utilized in diffusion models. With operations on latent patches by the transformer, this structure makes the Latent Diffusion Model possible. High-quality photos can be produced while efficiently employing CPU resources because of this method’s ability to handle image patches more effectively. A more natural video generation is thought to be facilitated by the use of this transformer, which is not the same as the stable diffusion that Stability AI revealed in 2022.
And also, it is important to keep in mind that their validation results show how scalable DiT is, which has a big impact on Sora’s realization. Scalability refers to the ability of the model to perform better as the depth/width of the transformer increases, thereby increasing the model’s complexity or the number of input tokens.
- Computational performance is measured in Gflops, equivalent to one billion floating-point operations per second on a computer. In this paper, Gflops are used as a proxy for network complexity.
- One of the metrics used to evaluate image production is FID (Fréchet Inception Distance), where a lower value denotes greater accuracy. By calculating the difference between the feature vectors of created and genuine images, it evaluates the quality of generated images quantitatively.
Kaplan et al. (2020) and Brown et al. (2020) have confirmed that this has already been observed in the field of natural language processing, confirming the critical features that underlie ChatGPT’s innovative success.
Capabilities of SORA
Up to now, we have discussed all the research that has affected the development of SORA and the technical functionalities of SORA. Furthermore, in the next chapter, we will pay attention to the current capabilities of SORA based on the report that has been published by OpenAI.
Image and video generation for the given prompt
As previously mentioned, Sora now creates videos in a text-to-video manner, where commands are provided via text prompts. But in addition to text, it is also feasible to employ pre-existing images or videos as inputs, as was clearly predicted by earlier research. This makes it possible for photos to animate or for Sora to visualize the past or future of a video that already exists.
Synchronization of Variable Durations, Resolutions, and Aspect Ratios
Mostly, NaViT allows Sora to sample videos in several formats: 1920x1080p widescreen, 1080×1920 vertical, and all in between. It may produce images for different kinds of devices at different resolutions.
3D consistency
SORA can create videos with dynamic camera movements, but it is unclear how the previously described study is directly related to this. People and scene objects move continuously through three-dimensional space when the camera moves in different directions.
The trajectory of Sora’s future
This article has been focused on describing the technologies underlying OpenAI’s Sora AI video generation system. After you read this article, you can clearly understand that Sora has already taken the world by surprise. It will undoubtedly have an even greater global impact if it is made publicly available and available to a larger.
Although this discovery is anticipated to have an impact on many facets of video production, it is also projected to progress beyond video to include other developments in 3D modeling. If that turns out to be the case, artificial intelligence may soon be able to produce graphics in virtual environments such as the metaverse with ease, in addition to producing videos.
In the near future, text-to-3D is also very likely, as Sora is currently thought of as “merely” a video generation model, but Jim Fan from Nvidia has hinted that it might be a data-driven physics engine. This raises the possibility that AI, from a vast amount of real-world videos and videos considering physical behaviors like those from Unreal Engine, might understand physical laws and phenomena.