Introduction
Climate change is among the biggest problems faced by humanity at the moment and political and social solutions can no longer be the only ones implemented. Over the past years, Artificial Intelligence AI especially Machine Learning, ML has great potential in contributing to the solutions to predict, counter or adjust negative impact of climate change.
Artificial intelligence – or more specifically, machine learning – is to make a computer learn from past experience and improve on itself without requiring anyone to code. This capability is already shown to be important in understanding large numbers of variables in the environment, in testing models for climate changes, and in determining solutions to mitigate emissions of carbon. Faster and more accurately than a human can accomplish, machine learning is providing scientific solutions to climate change by analyzing large amount of data.
In this blog post we will focus on how, and in what manner AI and ML are currently being applied in climate change research, key areas of application, benefits and limitations of such usage, and possible future developments. Knowing these technologies and how they are being used, will help us understand the part they play in building a sustainable world.
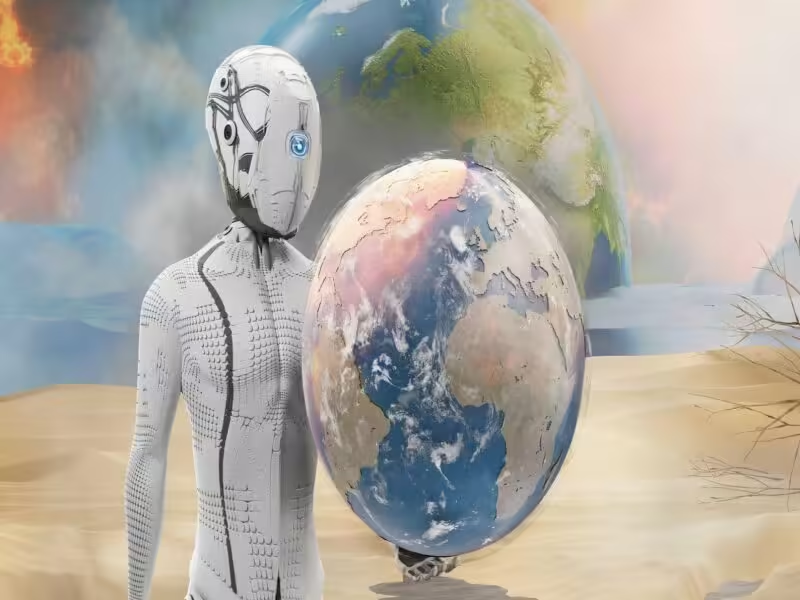
What is Machine Learning and How Does It Relate to Climate Change?
Understanding Machine Learning in the Context of Environmental Research
ML is one of the fastest growing fields in the field of AI that allows the computer to learn patterns with data to make prediction. The more data it receives, the more accurate its results and the insights that are to be provided to its users. In environmental applications, machine learning models are trained to study how the Earth’s systems work, how they will change in the future, and how they can be improved to reduce human contribution to climate change.
In contrast to the deterministic computational methods normally incorporated in computational models in which A set of procedures or operations that can be coded in a computer program are specialized and can accepted fixed parameters and deliver a sort of solution for them, there are the machine learning algorithms; such algorithms are capable of ascertaining regularities in data collected from the environment, and as new datum are gathered, the algorithms update their original learning to accommodate those new datum. This makes them good for the problems that are involved associated with climate change system as this involves very dynamic systems.
For instance, machine learning can use satellite imagery to determine the condition of the forests, estimate future crops production with regard to climatic parameters or simply estimate the concentration of carbon dioxide in the earth’s atmosphere. AI and climate change are getting correlated now as machine learning can not only model but also find effective solutions for the new environmental problem.
Why AI is Relevant to Climate Change Study
The climate systems are so complex that it is utterly difficult to study the correlation between climates and different climactic factors including temperature, humidity, water currents and gases in a climate system. With regards to this, the traditional climate models are often honed to failure within very short periods of time seeking to deliver approximately correct results for climate within a given huge number of data sets. This is where AI as well as Machine learning comes into the equation once again.
AI can deal with large input data such as imagery and sensor data, and other input data such as weather data. This kind of information can then be passed into the machine learning algorithms to analyze and reveal correlations that a human being cannot see when establishing climate models. Scientists also gain the ability to more easily and proactively model future climate scenarios with machine learning and therefore catalyze improvements in strategies for the control and management of climate change.
For instance, a machine learning model to predict changes in temperature over time from machine learning model where temperature data has been used to train this model based on various assumptions about greenhouse gases emissions. This predictive power enable policymakers say what may happen in the future due to climate change impacts and thus guide them in making decisions on the best approach to adopt in mitigating the impacts of climate change.
Key Ways AI and Machine Learning are Impacting Climate Change Research
Climate Modeling and Prediction
That said, one of the most promising areas of AI and machine learning application to climate research is climate modeling and prediction. Such early climate models involved complicated mathematical formula to represent the climate system and they existed when computation was a problem. Machine learning has helped to push this exercise to the next level and make it possible to develop better climate models at a faster rate.
Forecasting techniques are especially effective with improving the climate models. The second type, supervised learning, is used to teach the algorithms in the program historical climate data and predicted ones. Through entering coefficients such as level of CO2 in the atmosphere, temperature, or rainfall, AI can estimate future climate conditions which are important for global warming research.
For instance, DeepMind, an AI research lab owned by Alphabet, has used machine learning algorithms to forecast what ocean currents, which are determinative of climate, will do. With enhanced ocean realism in simulations, enhanced climatic realism, forecasted greater weather intensity and further sea-level rise, ensuing from an AI enhanced ocean simulation models.
Carbon Footprint Accounting & Reporting
AI is also very relevant meaningful a role in carbon footprint tracking and emissions control as well. Self-learning algorithms have made it possible for companies and governments to monitor emissions at the level of industries, city and even nations in the real-time approach. This information assists organizations in understanding their future developmental courses and determine ways of achieving lower carbon emissions.
Machine learning models can also identify patterns of energy utilization and then proffer how energy could optimally be conserved. For instance, AI applied energy management systems are now adopted at the industrial level to make changes at the energy demand level so that carbon emission could be curtailed greatly. In the same way, machine learning techniques are being employed in efforts to define patterns of poor supplies chain management adnation the manifests of transportation to produce the lowest possible amount of emissions.
Environmental Disasters Predicting
AI also playing a vital role in foreseeing and controlling the natural calamities like hurricane floods and wildfire. Modern researchers use machine learning algorithms to analyze meteorological data predicting natural disasters. Intriguingly, AI systems are capable of making predictions on the nature and place of the next storms, wind behavior, and ocean temperature, which are vital for disaster response groups.
IBM’s Green Horizon launched in 2020 creates the mix of machine learning in predicting the air pollution levels and carbon emissions throughout the regions so that governments and organizations that help in preventing and combating environmental calamities can make use of such data. Likewise, the likelihood of frequent wildfires is being predicted and other factors including; moisture in the soil, temperature, and wind patterns are being diagnosed using machine learning algorithms.
What is Machine Learning and How Does It Relate to Climate Change?
Understanding Machine Learning in the Context of Environmental Research
Thus, for further specification, Machine Learning comes under the subset of Artificial Intelligence as it allows the computer to discover the pattern and make a decision using their data without needing to be programmed to carry out each of the activities separately. In relation to the field of study of environment it implies that where the climate pattern or environmental outcomes are to be simulated or predicted the rules and the equations have to be fed into the system by hand, in machine learning systems the above processes are always self-enhanced with the data exposures.
In environmental science, data is big and normally complicated for regular analytic tools, and that makes machine learning useful for handling large amounts of data and information. Using these machine learning models, scientists can study satellite images, monitor the world’s climate, and make many other predictions when given numerous parameters – processes that were previously either unfeasible or took a very long time.
For example, in areas of deforestation monitoring, by training the machine learning models on satellite image data, these models can detect small changes in forest cover over time feasible for human detection. These models can identify problems that a human analyst may not see, and since the process is more time-sensitive, assessments of deforestation are timelier and more accurate, so that they can respond more promptly to help avoid further environmental degradation.
By the end of this research paper, the reader will understand Why AI is Crucial for Climate Change Research.
Climate change is a wicked problem, which requires analysis and tools beyound cognitive human capabilities. The climate of the Earth depends on a great many factors including amount of carbon dioxide in the atmosphere, sea currents, temperatures and even human activity. Earlier techniques of climate modeling have proven to be somewhat ineffective in dealing with such quantities of information.
This is where AI and machine learning come in handy, Machine learning models are capable of analyzing data from multiple sources and looking for certain trends knowing that standard models would not be able to pick up on these trends on their own. Such models can recreate potential future environmental scenarios, or forecast climate, or they can advise on how to solve climate change. With this capability, AI stands prepared to combat climate change since it provides forecasts while suggesting preventive measures.
For instance, machine learning has been applied to estimate the probable consequences of the Want of sea level due to the iceberg melting. Using previous temperature records, volumes of ice caps, and ocean data from around the globe, machine learning algorithms are able to not only say what is likely to happen in the future, but also to offer advice on how future rises in sea levels should be addressed. Such forecasts are essential for decision making in coastal city development and conservation, habitat management, and climate change mitigation measures.
Furthermore, it can help in making the ability to analyze the big data collected from remote sensing technologies such as satellite and several sensors useful, as the processed data would enable policymakers to make decisions at a fast pace by using the real-time data. This is especially important today owing to the climate change issue where early action is often crucial.
Key Ways AI and Machine Learning are Impacting Climate Change Research
Climate Modeling and Prediction
Climate models are now improving with the help of artificial intelligence and machine learning allowing for much better prediction of the climate in the future. Artificial neural networks can scan terabytes of climatic information from spaceborne remote sensors, weather stations, and dustbin archives and determine links between and among climate parameters such as ocean temperatures, greenhouse gas concentrations, and weather Fischer et al.
In classic climate models, an analysis of climate futures was a slow undertaking that demanded significant computer resources and takes time. However, machine learning allows climate models to analyse and simulate such datasets with high efficiency and increased precision. The advances in artificial intelligence based models has beneficially impacted the general weather prediction, climate change prognosis over long-term periods and even the specification of major climatic events as heat waves or hurricanes.
• AI-Powered Climate Prediction: For example, IBM has developed an advanced weather prediction tool called Deep Thunder that uses Machine Learning to give accurate weather forecasts right up to the quarter of the city. It also allows one to forecast storms and other climatic changes in detail and with much greater accuracy than paper-based models that are normally used.
• Advanced Climate Simulations: Researchers at MIT in 2023 used deep learning models to predict climate parameters regarding temperature and conditions how they might develop given the number of carbon emissions. It was more accurate than the older climate models to which people employed machine learning as a game-changer in future climate mapping.
Carbon Footprint Auditor and Reducer
AI is enabling the monitoring and minimization of carbon emissions at the industrial, city and national levels by analyzing data in real-time. Historically, carbon emission measurement is complicated; the results of this measurement are partial and imprecise. However, AI systems can always track emissions from different sources and adapt to energies in a way that lowers the average effect.
Real time predictions of inefficient energy usage are made by machine learning models with subsequent recommendations of alterations in industries. Such systems can also be programmed to control the lighting, Heating, Ventilation and Air Conditioning or HVAC installations of office complexes, industrial facilities and warehouses etc. Depending on the usage that is based on patterns of the particular area, fuel consumption can be minimized.
• AI and Carbon Emissions: Recently, if in 2020, google introduced an artificial neural network that caused a decrease in energy intensity of data centers by 15%. The AI system was able to dynamically control the cooling systems based on what machine learning recognized as the most efficient means by which to regulate temperature in real time, thus proving that machine learning can be used to spur used in energy intensive industries.
• Carbon Tracking: Other examples include; Carbon Clean sulfur uses Artificial Intelligence for tracking of emissions from industrial facilities in real time. Its technique entails the application of artificial intelligence to study emission and recognize potential areas of carbon capture and storage that will help to realize the carbon neutrality agenda.
Climate Change and Natural Calamity Prediction
Yet, one of the more vital areas where AI is applied to fighting climate change is in predicting environmental disasters. AI is also used in the daily predictions of natural calamities such as hurricanes, wildfires, floods, heat waves and so on, which are rapidly on the rise as a result of climate change.
Predictive models with the help of algorithms applied to large volumes of data collected from meteorological sensors or satellites, and so on identify potential precursors to adverse natural phenomena. These models can estimate such event’s occurrence and intensity, helping governments and organizations prevent and minimize consequences.
• Wildfire Prediction: For example, Google AI works with the fire management teams in California, to predict wildfires using data learning. With the help of such indicators as wind velocity, moisture of the ground, vegetation density, AI models can forecast how wildfires act, and people will know if the territories are at risk and need to be evacuated.
• Flood Risk Analysis: That same year, the European Space Agency (ESA) put into operation the system called Copernicus Emergency Management Services, which uses artificial intelligence to predict floods. This system utilizes satellite images and AI to forecast floods, giving humane emergency responders accurate data to aid in the rescue missions and minimize on the extent of property losses.
Machine Learning in Climate Change Mitigation and Adaptation
AI for Renewable Energy Optimization
While solar and wind power become more ubiquitous, the application of AI and machine learning is becoming more and more essential in enhancing the performance of energy technologies. By applying the machine learning, renewable energy can be properly produced, stored and properly distributed while minimizing wastage.
Wind energy; solar energy, they are not dependable sources of energy, you cannot really count on the wind to blow all the time as you cant on the sun to shine all the time. Another factor that has for long bothered experts in the field of renewable energy is timing, whereby supply cannot supply the demand. Automated control systems can also learn the prevailing real-time weather conditions and make decisions whether renewable energy is plentiful or scarce.
• Wind Energy Optimization: In wind farms, for instance, it is possible to incorporate the outcomes of data mining into accurate heuristic systems to identify the most suitable locations for installing the turbines and the most suitable method of operation. This provides the best efficiency of the turbines and is a function of changing the turbine speeds and angles in relation to prevailing weather conditions. According to the International Energy Agency, the use of artificial intelligence improved the productivity of wind farms by a maximum of 20%.
• Smart Grids and Storage: ;Machine learning is also useful in enhancing the storage and the distribution of renewable energy. Through intelligent command of smart grid facilities, the energy consumption and productive capacities can be balanced more proficiently and there is less need for fossil fuels. These grids take the help of machine learning for identifying the average energy consumption and then for making decision regarding storage of energy or actually putting energy into the grid. For example, the Tesla Powerwall uses artificial intelligence to manage energy storage in homes and the use of solar power depends on the pattern of consumption in a given home.
If AI is applied to improve supply-demand matching, the amount of energy thrown away to the air will decrease and renewable energy systems will be more efficient to reduce the use of fossil fuel and global warming.
The Roles Of Artificial Intelligence In Promoting Sustainability In Agriculture
The agricultural sectors have made a significant contribution to greenhouse gas emissions, but thanks to machine learning it makes agriculture sustainable. AI can be used to increase production per acreage, water and fertilizer efficiency and quality and keep the impact of farming on the environment minimal.
Farmers can use machine learning models to determine the characteristics of the soil, or weather predictions, or crop yields and make recommendation on how the farmer could optimize the use of these inputs. AI-driven precision agriculture enables the farmer to water crops, apply fertilizer or use pesticides where needed and when needed most – efficiently.
• Water Usage Optimization: Of equal importance, is the use of AI in water conservation. When there is a lack of water in an area, predictive algorithms may collect information on soil moisture and upcoming weather conditions in order to determine when irrigation is needed and in what amount so that farmers use water wisely. Per a record by The World Bank, Line Based Artificial Intelligence Irrigation Systems have cut down water usage by up to 50% in some areas and at the same time keeping crops healthy and the environment happy.
• Climate-Resilient Crops: The last application of AI is in breeding climate resilient crops. Machine learning models since they involve soil type, climate condition and crop genetics can help scientist determine the right traits for crops to cope with harsh weather conditions, pests and diseases. This will be important in transforming agriculture for climate change purposes.
Drones and satellite imaging that are propelled by AI are also used to assess crop health and other challenges such as pest invasion or diseases and nutrient paucity. Such real time monitoring assists farmers in preventing problems from getting out of hand where the yields are increased and there is less use of pesticides.
The integration of AI in Urban Planning for Sustainability
Substantial emissions are also generated by urbanization whereby cities contribute 73% of global emission. Nevertheless, AI is taking a central role in developing tuned and resilient cities that are more capable of managing effects of climate change.
The technology of machine learning is being applied in developmental planning to ensure that energy and resources are only used optimally. AI can forecast the energy consumption in cities, enhance waste management and design a transport system that releases less energy.
• Smart Cities: AI can contribute towards smart city development since it only requires the installation of sensors and further utilization of a machine learning model to analyze energy consumption, traffic flow and environmental status. Using big data to improve these factors in real time benefits kindergartens, elementary schools, middle schools, high schools, colleges, and residents of the city by decreasing congestion and air pollution.
• Sustainable Architecture: Machine learning is also used in the assessment and design of green buildings or efficient buildings. AI can replicate the behaviors similar to the effectiveness of the building in which or how much lighting, heating, cooling is required at various time of the day or month. This means that architects can now design structures that use less energy in an effort to lower the impact of carbon emission within the construction field.
AI is also deployed in the transport industry where public transport is being improved. Traffic and rider data, as well as weather and other environmental influences, can be tapped by machine learning to dutifully arrange bus and train time-tables for pollution-free cities. Electric cars are also increasingly incorporated into city layout, and AI is assisting to arrange the networks for charging as well as for moving the existing cars.
The Future of AI and Climate Change Research
What’s Next for AI in the Fight Against Climate Change?
The promised benefits of Artificial Intelligence (AI) and data learning for climate change cannot be fully realized at the moment has started to revolutionize branches and disciplines. However, even more hopeful is the future of AI in climate change research. Over time, AI technologies in Clemson will help scientists and policymakers to understand weather patterns to improve the use of natural resources and find appropriate solutions to the ecological problems.
In the coming years, the evolution of AI is expected to bring several key developments that will further enhance climate change research:
• Integration of Quantum Computing with AI: Of the most promising ways for the future, one has to classify the integration of quantum computing and the AI field. Quantum computing can take volumes of information that could be processed in quantitative analysis and process it at a rate faster than that of today’s computers. If combined with AI, it may bring a radical change to climate modeling by generating complex future scenarios for the environment incomparably faster and more accurately. This could lead to improvements in real time and high accuracy, faster decision-making of climate change impacts.
• More Advanced Climate Models: As we note, the use of machine learning in climate models will become much more enhanced in the future, and we will be able to observe such complexities as regional climate, or other aspects of climate, extreme weather events, and any ecological changes in the climate models with much more precision in the future. Such models will not only be more precise in their prediction but also capable of incorporating an increased number of variables – from people’s behavior to policies of the surrounding environment.
• Real-Time Monitoring and Decision Making: AI will persist to expand the methods of advanced environmental tracking that enables instantaneous tracking of the health of the world environment. Data from sensors and satellites can be instantaneous in which they can be analyzed by an AI system in order to generate recommendations to be taken in order to mitigate or adapt. For example, potential risks connected with climate change could be monitored in real time: AI might predict a heat wave, floods, or other unfavorable conditions and suggest how it is best for cities to adapt.
• AI-Driven Carbon Capture and Storage: Carbon capture technologies which have the goal of capturing atmospheric CO2 emissions and storing them appropriately remain critical for attaining carbon neutrality. AI will of course be central in the development of these technologies . Machine learning can also be used later to fine tune the efficiency of molecular capture, determine optimal locations for the deposition of captured CO2, and enhance the economic viability of such operations.
• Collaborative AI Research: The future of AI in climate change research will not be gated to one researcher or any organizations. These kinds of projects will help create a global ecosystem of countries, academic research and tech companies who can work together to pool the kind of resources and data necessary to create more credible, solid climate models. As open source tools and even datasets become more easily accessible and more common, more researchers around the world would be able to help fight climate change through their work, leading to faster rates of development in the years to come.
Recent Development in AI Applications for Climate Change Mitigation
It is crucial to understand some of the current and potential applications of emerging AI methodologies in climate change research as of now, they have started being evident and will most probably proceed to grow in the next few years. Some of these include:
1. AI in Geoengineering: Despite being considered currently as too futuristic, geoengineering may well be included in future strategies as a tool to fight climate change. AI can by means of simulations analyze the feasibility of geo-engineering solutions like SRM or CDR to reduce global temperatures. It is still doubtful whether geoengineering actual impacts should be actually used but the simulation of it with AI will be indispensable in decision making about them.
2. AI-Powered Circular Economy: This reoccurring problem is one of the most creativity solved using AI for climate change solutions through supporting circular economy. Circular economy is a closed-loop system, whereby resources are used again, recovered and given another use. This leads to making recycling benefitted, recognizing valuable products apart of the waste management tires, and even estimate demand for recycled products of the circular economy system.
3. AI-Enhanced Climate Finance: AI analytics are also rapidly gaining importance for the practice of climate finance as well. AI can provide general information of climate risks in different portfolios and inform investors and organizations the potential of investing in green technologies. They also capable of processing the ESG data and giving much more precise cues about climate-risk related financial opportunities and threats.
4. Integration of AI in Policy Development: We also notice that AI is being integrated into the process of creating climate policies. Through the use of Machine learning it is possible to conduct a rational examination of the effects any choice of the policy has to make; forecast the results of its work and simulate the results of different strategies. As a tool for optimization, AI can run a detailed time simulation through which it will be possible to determine what effects some policy actions – be it, for instance, carbon pricing or renewable energy incentives – will have on emissions levels.
Integration of Scientists, Governments, and Innovators of AI
The next phase of using artificial intelligence in fight against climate change will only be possible depending on the level of cooperation in the governments, researchers, and such technology companies. The issues we face today are much larger than anyone’s imagination can grasp and the solutions have to be international in nature and one such tool which can straddle across sectors is AI.
• Public-Private Partnerships: Sadly, some of the more interesting AI-driven climate initiatives are already being developed with governments, research institutions, and private entities. For example, Microsoft and Google aim at reaching the state of carbon neutrality in the framework of cutting down own carbon footprints with the help of AI. Governments are also waking up to the necessity to address climate change and are thus coming up with different polices and incentives to encourage the use of AI. In effect, the adoption of AI solutions requires partnership agreement between the governments and the innovation departments established to enhance thee technologies to suit the needs of entire nations.
• International Cooperation on Climate AI Research: Climate change is an issue of global concern and, therefore, needs cooperation at that level. As a result, nations globally need to collaborate and exchange more data, research and AI tools, aimed at resolving the global climate crisis of increased emissions and climate change impacts. More and more UNFCCC and other comparable institutions are beginning to accept AI implications of climate change and provide encouragement for citizens to collaborate across national borders.
• Citizen Participation in AI Climate Solutions: Continuing this, there’s another equally fascinating trend in climate change solutions – the involvement of citizens and the public through citizen science and use of AI. Those demanding and complicated instruments will become globally available to folk, and those individuals can insert data, get competent measurements of carbon footprints, and apply climate research. This democratization of AI can lead to a more participative approach towards climate intervention as citizens and small scale business are given tools to start taking some level of climate change interventions.
Conclusion
Due to the increasing effects of climate change all over the world it has become important for researchers and innovation to adopt the use of artificial intelligence and machine learning. AI has been established as an impactful innovation offering scientists, politics, and industries means for gathering and processing enormous volumes of climate data and designing and implementing potent means for slowing down the climate change.
AI is increasingly drawn into climate change research from refining climate models & carbon emission log to maximizing renewable energy, & leveling up agricultural sustainability. Artificial intelligence has presented itself as maybe the most crucial method of combating climate change due to its ability to provide answers to inquiries that, before, would have been unimaginable. These technologies have helped us not only to predict changes in the environment, but also to adapt them to our advantage, make energy efficient, reduce emissions and promote sustainable development.
But as with all things, it is worth also stating the facts about hurdle that AI is going to face in the fight against climate change. Solution concerns that include lack of adequate data, presence of bias in learning machines, as well as the computational cost of AI models have to be dealt with. Moreover, the appropriate applications of the AI technologies will be the important factors that determine that the main goals of the environment and society’s improvement will be attained.
Furthermore, harnessing the potential technology brings has great opportunities on the future of both AI and climate change. Suddenly incredible technologies such as quantum computing, AI powered carbon capture, and real time environmental monitoring can turn climate change management into a first rate, AI informed, technological challenge. AI will need to become firmly established in climate policies as well as the formation of international cooperation of governments, scientists, and private companies, who are inventing technologies that will allow such advancements and real change.
When it comes to tackling climate change, AI and machine learning are not a resource – they are assets which will assist humanity in understanding the current climate and its future trends. Yet these technologies will help us to create a sustainable environment in the future for the generations to come.
References
- “AI and Climate Change: The Role of Machine Learning in Environmental Research”, Nature
- This article discusses how AI and machine learning technologies are transforming the way scientists approach climate change research, highlighting key applications in predictive modeling, disaster forecasting, and more.
- “AI for Predictive Climate Modeling”, MIT Technology Review
- This source provides insights into how machine learning models are being used to simulate and predict climate patterns more accurately, offering a detailed view of AI’s role in improving climate predictions.
- “Harnessing AI for Climate Change Solutions”, Science Direct
- This paper explores various AI applications in climate change solutions, from energy efficiency and carbon emissions tracking to waste management and sustainable agriculture.
- “AI and Renewable Energy: Unlocking the Potential”, International Energy Agency (IEA)
- This report discusses how AI is optimizing the use of renewable energy, particularly wind and solar, through better energy forecasting, smart grid integration, and predictive maintenance.
- “Machine Learning in Precision Agriculture”, The World Bank
- This reference covers how machine learning is being used in agriculture to improve water usage, crop yield, and soil health, contributing to sustainable agricultural practices that help mitigate climate change.