Introduction – The Role of Meta-Learning
With the rising applications of artificial intelligence (AI) in various aspects of our lives the problematic of using AI ethically is getting to prominence. From cars that drive themselves to security cameras that identify faces, the assessment of important social issues is being handled by artificial intelligence systems leaving little if any room for human input. This brings us to a critical question: Is it possible for AI to find a solution to some of the ethical questions which it according to Robinson creates? If so, what part does meta-learning contribute to such a situation?
Meta-learning also known as learning in learning is a new generation learning paradigm into AI applied that enable systems to learn on their own gradually. In case of the ethics of artificial intelligence, it bears the potential of allowing machines to decide and choose independently, things that are right, moral and ethical. But is this truly possible? In this article, the author will review ethical issue in AI, how meta-learning can solve it, and what are the open issues.
To get more familiarized with this exciting field, let’s explore what constitutes AI’s ethical issues first.

What Are AI’s Ethical Dilemmas?
The Complexity of AI Ethics: Why It Matters
AI is already been predicted to disrupt every sector ranging from the medical sector to the financial sector and even education sector. Nevertheless, since AI systems are gradually turning into self-driven systems, they are also inclined to face a range of ethical issues. Such choices occur because AI systems choose courses of action important in human life and the chosen course may not meet humane ideals.
Some of the most pressing ethical concerns in AI include:
- Bias: Most AI learn from big data that, if contains biases, then the AI would reinforce those biases or even escalate it. For instance, facial identification software demonstrated poor performances for people of a color, and, therefore, may be racially prejudiced.
- Fairness: This suggests that many AI systems can unconsciously be prejudiced in a way that serves some populace and penalizes others, and thus some AI outcomes may not be fair to some parties. For example, if algorithms are applied to hiring circumstances, then the results could possibly be prejudiced to a certain group of workers regardless of the original intent to avoid it.
- Accountability: In case an AI system is wrong or even damaging, should have anyone be accountable? To ask the question, is it the use of the developers, or the users themselves, or even the AI? These questions are very important when it comes to other line systems like self-driving cars.
- Transparency: It is often seen that many AI operating systems are ‘black boxes”, which do not reveal how they arrived at the decisions that they have made. This absence of public record – let alone public explanation – undermines faith and responsibility, particularly where the stakes are greatest.
It is, therefore, crucial to resolve the presented ethical issues. If AI-driven systems are going to make societal decisions, they have to be fair, non-bias and their processes have to be transparent. But how can this be done, particularly as the use of AI advances?
Ethical decisions: how AI systems approach it?
AI systems act on the basis of the data that they received and the algorithms that were used on them. In other words, these systems rely on patterns in the given data for arriving at decisions or to make some predictions. But in case of ethical decisions, the process is a little complex.
For instance, let’s imagine an auto-mobile car which is designed for avoiding an accident all of a sudden requires to be involved in an accident. The app in the car must choose between steering the car into the pedestrians or continuing the drive and crashing into a barrier inflicting pain on the passengers. The movie’s choice described in this scenario is known as the trolley problem based on the issue named so. However to humans the decision making may seem simple and straight forward but programming an AI to reason about even these basic moral dilemmas is a big deal.
One such example can be identified from the AI-based Health Care facilities. There is a day that different formulas are being employed in arriving at decisions that concern the lives of patients within the hospitals, for instance, decisions on who should receive transplants. These decisions have to be made understanding human values, priorities, and fairness and these are domains AI fails at.
Such choices are made by using certain principles of operation or treating them as patterns once learned, and thus do not apply the moral reasoning which people do when resolving an ethical issue. This is where meta-learning could come in handy, and help to solve the problem.
Meta-Learning as a Component in AI’s Moral Agency
What is Meta-Learning?
Meta learning also referred to as learning to learn is a concept within machine learning whereby an AI system learns how to learn. Instead of being learned on one particular task, meta-learning allows AI to adapt the way it learns depending on the previous experiences. In other words, it lets the machine learn at a faster rate by proactively changing up its style depending on the problem. Traditional forms of AI are trained on one dataset and function optimally as long as they are operating in the scope of that particular dataset. Finance to education. However, as AI systems become more autonomous, they also face a variety of ethical dilemmas. These dilemmas arise because AI systems often make decisions that affect people’s lives, and those decisions may not always align with human values.
How Do AI Systems Face Ethical Decisions?
AI systems make decisions based on the data they are trained on and the algorithms that guide them. In essence, these systems rely on patterns in the data to make predictions or take actions. However, when it comes to ethical decisions, the process becomes more complicated.
For example, consider an autonomous vehicle faced with an unavoidable accident. The car’s AI must decide whether to swerve and risk hitting pedestrians, or stay on course and crash into a barrier, potentially injuring the passengers. This scenario is a classic example of the “trolley problem,” a well-known ethical dilemma. While the decision may seem straightforward to humans, programming an AI to navigate such complex moral choices is much more difficult.
Another example can be found in AI-driven healthcare systems. Algorithms are being used to make life-or-death decisions about patient care, such as determining which patients should receive organ transplants. These decisions must be made with a deep understanding of human values, priorities, and fairness—areas where AI still struggles.
AI systems make these decisions based on predefined rules or learned patterns, but they often lack the deeper moral reasoning that humans use to navigate ethical dilemmas. This is where meta-learning could play a crucial role.
Can meta-learning be of great assistance to AI in solving problems related to ethical questions?
Now that we understand what meta-learning is let’s think how this approach could assist AI systems experiencing ethical issues. One of the effectiveness of AI is flexible learning based on previous data encountered hence might be better placed to tackle intricate moral obligations.
Meta-learning is useful in that it let’s AI become generalized in its knowledge. For example, an AI business system designed to handle one ethical decision can transfer that decision-making approach to a completely different scenario. Here’s how this could work in the context of AI ethics:
- Self-Adaptation: From the previous ethical decision, meta-learning could facilitate the feeding of AI systems. For example, if an AI system employed in a particular organization decides on an ethically questionable solution (for instance, hiring one group and passing on the other), the AI system will self-correct on the guidelines used the next time a similar decision has to be made.
- Ethical Evolution: In the long run, the Ethical AI system may help to optimize the action of ethical decision making through the feeding of new data as well as new experiences. AI connected to real environment may grow in stages and get a notion of ethical approaches different from but all in line with the existing moral of mankind.
- Contextual Understanding: Meta-learning makes AI to be more adaptive in the ethical contexts of its responses to present the nuances quite effectively. For instance, a self-driving car should make different decisions depending on surroundings, weather conditions or the level of emergencies. It is suggested that through meta-learning it will be able to better respond to such complex situations.
- Avoiding Bias: Meta-learning could also help AI to detect and minimize its bias own to itself. AI would be able to avoid biases because, just like any other human, it was capable of realizing mistakes that it had done before and steer away from them.
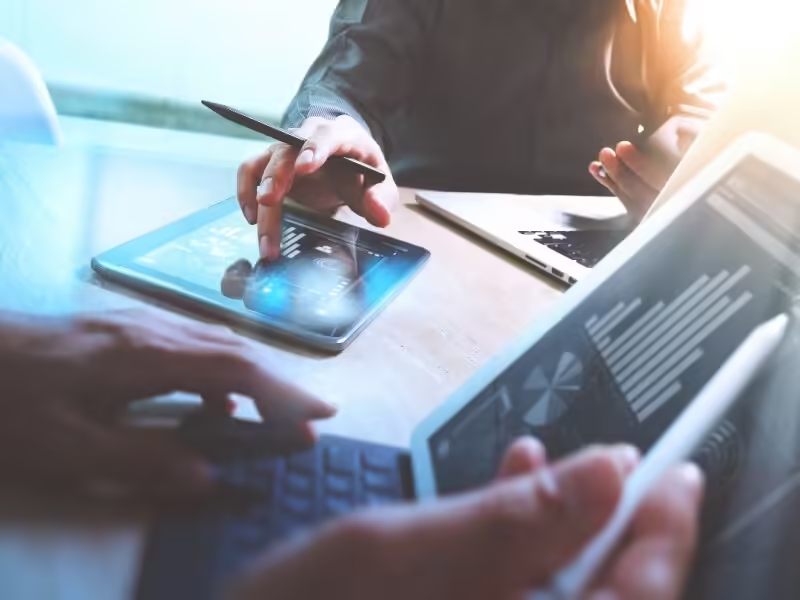
The Advantages of Meta-Learning in Overcoming Ethical Decision-Making
It is therefore feasible to solve AI ethical issues using meta-learning. Some of the most important benefits include:
- Flexibility and Adaptability: AI systems could improve over time, including developing solutions for new ethical problems, which would keep them suitable and ethical correspondingly to people’s changes over time.
- Self-Improvement: It was claimed that in due course, AI might be ‘shedding’ incremental improvements in ethical decisions based on experience. The eventual of self- improvement would diminish dependency on human interaction and operational involvement across the process which would empower AI to be more proactive.
- Bias Reduction: It was suggested that meta-learning can be used to enable the AI system to ‘learn fairness’ so that it will not make discriminative decisions.
- Scalability: Meta-learning’s potentiality describable from a plethora of ethical questions means that meta-learning can be scaled in various applications across many fields including health, law enforcement and education.
Overall, meta-learning holds the key to offering AI the means of addressing its ethical issues that will automatically enhance its availability, impartiality, and accountability for its actions.
Current Limitations of AI and Meta-Learning in Ethics
Challenges in Applying Meta-Learning to Ethics
Captured by the general idea of meta-learning that promises to develop generic knowledge for a broad class of AI environments to solve the ethical issues, there is a list of challenges to discuss to harness the approach in the context of ethical decision-making. All these complications stem from the aspect of complexity of AI systems and inherently diverse challenging nature of training a system to comprehend the ethical practices as embraced by human beings.
- Defining Ethics for AI: In fact, one of the major challenges in applying meta-learning in ethics is the fact that ethics as a course is highly nuanced and cannot be reasoned as simply as other courses. When is comes to moral criteria, there is wide variety depending on culture, society, and person. Selecting an ethical approach suitable for an AI is an issue. Should AI decisions be taken based on utilitarianism, deontology or teleology? Selecting on the single ethical system that AI should be trained in and then implement is not easy.
- Technical Limitations: Further, meta-learning entails that AI systems have to handle large data corpus and make decisions on the same data. However, teaching the system to generalize well across various ethical incidences is a process that is extremely complex. Meta-learning algorithms have to identify a range of ethical concepts and to learn how it is possible to use these concepts in practice; and this is a far from being a recognition of certain data patterns.
- Data Dependency: However, for meta-learning to be of benefit meta-learners require good data that represents the general ethical problems and the systems have to be able to access such data. This means that if the data used to feed AI systems is incorrect or limited in some way or even contains racy bias, AI will also be wrong or limited. Due to this, it is necessary that the training data is composed in the best way possible and in a good representation of various points of view.
- Complex Decision-Making: Sometimes, ethical decisions always involve the clash of ethical values, and people cannot be certain which way is ethically correct. For instance when an autonomous vehicle is approaching a crossroad and knows that it can cause an accident, the ethical decision about the accident can change depending on the lives of the people who may be involved and the values they hold dear. Nudging AI for those high-staking, analytically intense decisions via meta-learning of course, is another grand challenge.
Ethical Issues with Using AI as a Solution to Ethical Problems
Less obvious is the question of whether AI is capable of addressing these problems or whether it is even right for AI to do so. While meta-learning may provide AI with the tools to adapt and learn from its ethical decisions, there are significant risks associated with allowing AI to self-regulate its ethical framework:
- Loss of Human Control: Some quarters have been worried that perhaps the AI systems being developed today might over time develop into systems that are not compatible with human values. However well-meaning an AI system may become it has the capacity to learn and to modify its ethical decision making process and this could lead it to make decisions which are out of control of or beyond the understanding of most people.
- Accountability: When the task of decision-making involves ethical issues, their management in AI systems raises the problem of liability in case of an undesired outcome. Who is accountable for the decision in case an AI system results to causing an injury to a person? Which should answer it: the developers of the system, the agents who implemented it or the AI? Some of these questions are yet unanswered:
- Lack of Transparency: Deep learning techniques also present new limitations to the liability of artificial intelligence systems because these systems are frequently’ black boxes; the original developers of the AI systems cannot always comprehend the rationale of AI decision-making processes. Such practices can be called ‘nontransparent,’ and they prevent people from believing AI’s ethical decisions and questioning the fairness and responsibility of an automated decision made.
- Ethical Dilemmas of AI Decision-Making: There’s definitely a risk here: if one tries to build AI that can state and solve an organization’s ethics, one might be creating an entirely new set of ethical problems. For instance, if an AI system learns that some decision is in the people’s best interest, something that can improve the quality of life for most folks it can rationalize actions that are prejudicial to a minority group thus offing fairness.
Which Unintended Consequences in AI Ethics can Meta-Learning Contribute to?
As much as meta-learning would improve ethical decision making for AI’s, it comes with the possibility of unforeseen negative impacts. Since AI is expected to make its decisions based on past action, it means that the previous actions could further deepen biasness in its decisions. For instance, if an AI system characterizes some ethical considerations as being ‘better or preferable’ than other considerations (for instance deeming efficiency as preferable over fairness), the AI system will, time and again, make decisions based on this preference.
Furthermore, because meta-learning is still a developing field, there remains the possibility for both realistic and misrepresentative learning, where unpredictable models may change the outcome of the tasks that AI systems perform. A system that was built with the principles of making ethical decisions in one ethical framework can switch to another ethical framework without the interference of the human to make ethical decisions that may not be ethical as per the society standards or norms.
Also, meta-learning could help keep ethical decisions stagnant.
Perhaps, the worst of all is that if training is performed using past decisions as an example, these new AI systems and tools can often use them as the only source of decision making and therefore, they will not be capable of developing new ethical approaches to manage new unforeseen situations. In such fast-developing areas as AI, such halts may postpone the ability of the systems to respond to new ethical issues, which arise in the course of utilization.
Conclusion
Thus, meta-learning Calvin will be an important approach to help AI grapple with its ethical issues by learning from the previous incidents and adjust for a better result in the future. But there are going to be significant problems and potential dangers in extending this meta-learning to the field of artificial intelligence ethics. The need to undergone five thought-provoking and very important stages seems clear before artificial intelligence can come up with its own ethical resolution: developing the ethical framework that can be utilized universally, eradicating technical barriers, and solving the issues related to accountability and transparency.
Thus, the further advancement of AI and the deepening of meta-learning research require thinking through the exterritoriality of the process of assigning greater control over ethical decisions to AI. Human intervention will, presumably, always be required in order to ensure that AI behaves in the way that is compliant with the culture and norms we choose to adhere to.
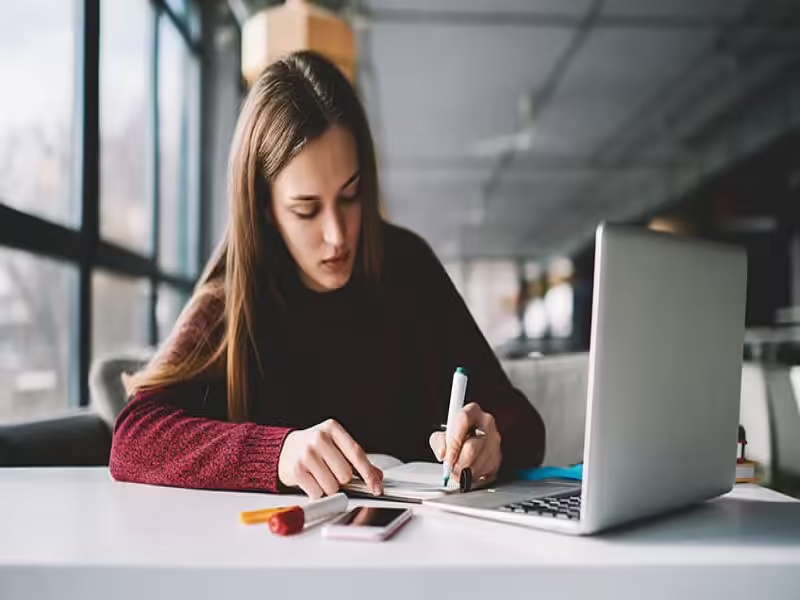
References
- Russell, S., & Norvig, P. (2016). Artificial Intelligence: A Modern Approach (3rd ed.). Pearson Education.
- Floridi, L. (2018). The Ethics of Artificial Intelligence. Oxford Handbook of AI Ethics.
- Lake, B. M., Salakhutdinov, R., & Tenenbaum, J. B. (2015). “Human-level concept learning through probabilistic program induction.” Science.
- Vincent, J. (2020). “AI and ethics: Why we need to consider the dangers of autonomous systems.” The Verge.
- Amodeo, G., & Marini, F. (2020). “Meta-Learning and AI Ethics.” AI and Society Journal.
- European Commission. (2019). Ethics Guidelines for Trustworthy AI.