AI has emerged rapidly, and in its wake is the cutting edge concept of deep learning. As the creation of complicated and advanced neural networks, deep learning provide new vigour for different subjects including image and speech recognition, drug discovery, and self-driving vehicles. Here, in this ultimate guide to deep learning, we will attempt to explain what this concept is all about, how it works, where it can be applied today, and where it might be used in the future.
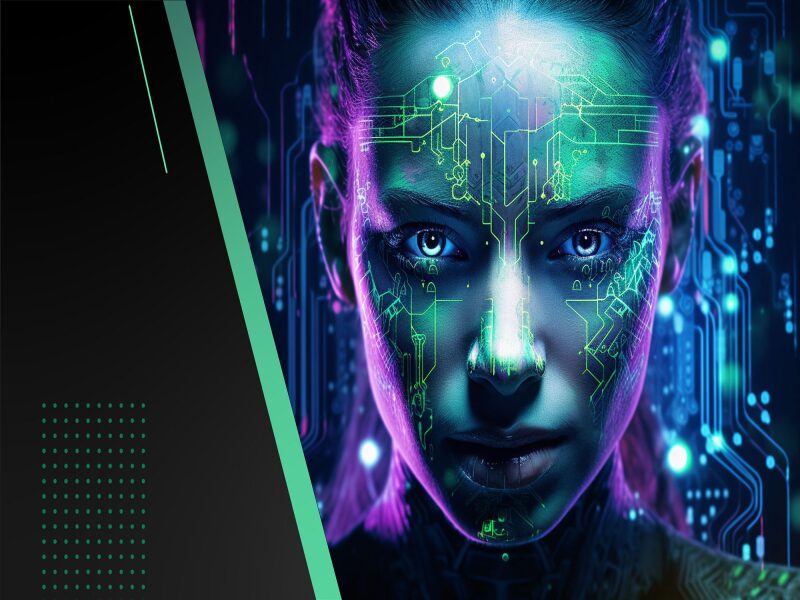
Understanding Deep Learning and Neural Networks – The Power of Deep Learning
Now that we are ready for the tour of the world of deep Learning let us Ensure that we have the foundational concepts of the transformative technology clear to us.
What is Deep Learning?
Concrete, deep learning is a part of machine learning that utilizes artificial neural networks to train the algorithms on large sets of data, and then make sound choices. Compared to most other algorithms under the machine learning domain, it automatically identifies the higher-order representations in data and models complex patterns that cannot easily be observed. This makes it possible for deep learning models to perform the task up to the expectation of how a model designed for a particular task would do it.
What are Neural Networks?
In the center of deep learning there are so-called neuronal networks, which are computational models based on the structure of brain neurons. They are layers of nodes, or artificial neurons that function cooperatively and perform or transfer information. This kind of neuron takes input signals, and processes some computations, then the result of that computation is to produce an output signal to the next layer. By such a structure, there is the production of multiple levels of data patterns and representations within the neural networks.
How Does Deep Learning Work?
The training of a deep learning model is a continuous manner whereby large amounts of data is feed to the model with corresponding labels. After that, the data is used by the model to infer the patterns that exist in the data set. This learning is not a one trial process but the process of adjusting internal parameters of the model to maximum parameters.
Another very important method that is used is called backpropagation, which allows the model to get the error of the prediction needed to be made, and back it towards each layer in the neural network. The said weights and biases when changed on the neurons and the interconnections between them make the model better in predicting results.
Key Advantages of Deep Learning
Deep learning offers several key advantages that set it apart from traditional machine learning approaches:
- Ability to handle complex data: Hence deep learning models are well adapted for handling big and complex data such as images, videos or even plain text itself. They can come with statistically significant features from raw data without having to manually extract them.
- Automation of feature extraction: Kyu and others for instance, traditional machine learning algorithms call for human intervention for the feature engineering process. However, in the above work, what deep learning was able to do was this in an automated way so that the model would learn which features were most important on its own.
- High accuracy and performance: The proposed deep learning models are favorable and innovative and have exceeded human performance in tasks such as image recognition and natural language processing.
- Generalization capabilities: The type of learning that is used in the deep learning models allow for the models to have flexibility in a way that the model can work differently according to the situation and the challenge.
The stated advantages make deep learning one of the most efficient approaches to handling the complex issues, as well as designing a number of developments in various fields.
In the next section, we will discuss each of the various applications of deep learning in detail according to the respective domains and industries.
Applications of Deep Learning: Transforming Industries
Many fields and domains have incorporated deep learning thanks to it outstanding performance, changing the way people deal with technology and analyze different issues. Now let’s look at some of the most well-known and influential realizations of deep learning which influence the world we live in.
Image and Video Recognition
The last few years have seen deep learning bring about a huge change in the way image and video recognition is done. Starting from detection and recognition of the objects and faces to scene laboratories and various anomaly detection methods deep learning algorithms have enhanced numerous uses.
- Object Detection and Recognition: Using deep learning models, it is possible to easily detect objects in images or even within videos, creating possible areas of use such as self-driving cars, surveillance, or augmented reality.
- Facial Recognition: In one’s face, computer vision programmers based on deep learning can recognize individuals with great accuracy for use in biometric security, monitoring, and advertising.
- Video Analysis: It is possible to recognize actions, make scene summaries, and even apply filters to videos in real-time as the deep learning algorithms sort through the torrents of streaming video.
NLP: an acronym that stands for Natural Language Processing.
Deep learning made huge strides in the field of natural language processing (NLP), the branch of artificial intelligence that attempts to replicate the manner in which people use and understand language. NLP made great strides in language translation, speech and text emotions, chatbot and even in writing on its own alongside the deep learning models.
- Language Translation: There exists a capacity of the deep learning translation system, which utters and translates text and speech from one language to another, hence improving on the communication barriers.
- Sentiment Analysis: Text classification can use deep learning mode to discover the sentiment or the emotional point of view of the text stream leading to intelligence apps in fields like social media, customer feedback and brand reputation.
- Chatbots and Virtual Assistants: AI interfaces such as deep learning based chatbots and virtual assistants empowers them to answer queries in natural language and can take up an individual’s customer service and query answering and other related tasks.
- Text Generation: Regarding further development of new deep learning models, there is a number of potential uses – writing, story writing, creating artwork, and even musical compositions.
Speech Recognition
The translation is made possible by the speech recognition capability that has been made possible by deep learning. This has led to development of some related ones like voice assistant, voice transcription, and voice commanded devices.
- Speech-to-Text Conversion: It can transcribe speech subsequently making its potential applications in speech to text dictation, transcription and real time text relay for the deaf or hard of hearing.
- Voice Assistants: Smart technologies such as Siri, Alexa and Google Assistant employ DL based speech recognition for voice input to augment voice control and ergonomics in technology devices.
Recommendation Systems
It has become very easy to recommend products, movies, songs and posts to the users by deep learning that has influenced the recommendation systems greatly and widely ranging from e-commerce sites to music and video streaming services and social media platforms.
- E-commerce Product Recommendations: Automated deep learning systems can make decisions on a user’s behavior, purchasing habits, and product characteristics in order to recommend specific products to them: boosting sales and loyalty.
- Content Recommendations on Streaming Services: Conventional recommendation systems involve analyzing the user’s preferences, history of viewing or listening, and metadata about the content to suggest movies, shows or music that courses can recommend such content.
- Social Media Content Recommendations: Consequently, deep network learning can decode user interaction patterns, preferences and friendship circles to recommend content to users through the social media platforms.
We now proceed to the other areas as important as healthcare such as deep learning in self-driving cars for the next topic.
Healthcare
Artificial intelligence specifically has advanced through the help of deep learning in the healthcare system, changing many areas of the patient’s treatment, diagnostics, and more. Another advantage that have made it possible to solve some of the world’s most complex medical challenges include medical imaging, differential diagnosis and drug discovery and development.
- Medical Image Analysis: Real life use of Deep learning is a great example, this can interpret medical images such as X-rays, CTs, MRIs among others with high end precision. They are capable to diagnose tumors, find out inconsistencies and help radiologists to make quicker and more precise diagnosis.
- Disease Diagnosis and Prediction: It is also possible to apply deep learning models for analyzing patient information to predict the likelihood of such diseases in future depending on the past medical history status, manifested signs and symptomatology, genetic data. It can contribute towards early diagnosis of such conditions prior to arrival of fatal stages.
- Drug Discovery: Deep learning is a crucial component in the fast and efficient identification of drug candidates and the prediction of their effectiveness as well as the fine-tuning of the drug’s design. They may lead to improvement of the existing treatment and discovering some other better treatment to diseases.
Autonomous Vehicles
Deep learning self-driving cars is one of the most promising and captivating application of deep learning technology. These vehicles use sensorial systems, cameras and deep learning to detect neighboring vehicles, road signs and traffic signals and then make correct decision about when to accelerate, decelerate, turn and stop among other maneuvers.
- Perception and Scene Understanding: Self-driving automobiles employ deep learning to differentiate the environments around them and the scenes inside them. Among the tasks that accompany such an annotation process, there is an object detection, possible lane detection, or even pedestrian recognition.
- Decision-Making and Navigation: In particular, the perceived information is analyzed by deep learning algorithms and decisions on changes in acceleration, braking, steering and lane changing are made at the moment. They also perform traffic routing and avoid various hurdles.
- Safety and Efficiency: Self-driving cars utilize deep learning as one of the key technologies that guarantees that the car will run appropriately on roads. Thus, deep learning allows perceiving the environment with high accuracy and making adjusted reaction to avoid accidents and improve the traffic organization.
There is a rich list of deep learning application areas, and it is rich only in terms of concepts and feels to be even bigger in the nearest future. Deep learning has also been applied in improving healthcare, transforming transportation, powering recommendation systems, and natural language understanding to mention but a few applications that are already in the market and several other discoveries in deep learning are forecasted to come to the market in the near future. In the subsequent section, the aiding instruments and models that facilitate the construction and implementation of deep learning solutions are discussed.
Deep Learning Frameworks and Tools
Deep learning has grown rather quickly due to the newest frameworks and tools that help ensure that the process of creating, training, and deploying is made easier. These frameworks enable a diverse environment of libraries, APIs and even pre-trained models waiting for the researchers’ of developers’ intervention. Now let’s focus on some of the most prominent deep learning frameworks and tools.
TensorFlow
TensorFlow, developed by Google is perhaps one of the most widely used, open source deep learning frameworks, which offer flexibility at scale and rich community support. That is why it offers the entire enabling environment for designing, training and creating deep learning models and others. TensorFlow’s key features include:
- Tensor-based computations: TensorFlow uses data structures called tensors that are in fact multidimensional arrays, suited well for numerical computations and optimization across related devices.
- Flexible architecture: In TensorFlow, you can declare a data and computations which state a particular graph of computations in the neural network model. Such flexibility allows you to create and develop various model designs capable to be tested with models.
- Eager execution: One of the big changes that were made in TensorFlow 2.0 is eager execution, with which operations are executed instantly and the results can be viewed interactively which greatly facilitates debugging and experimentation.
- Deployment options: TensorFlow models can run on diverse accompanying hardware such as desktops, servers, mobile, and embedded gadgets.
- Large community and resources: Currently TensorFlow has a very active and creative community, that has been developing a lot of resources including documentation, examples, and even ready-trained models.
PyTorch
PyTorch is a deep learning toolbox created by Facebook’s artificial intelligence research group. The features include dynamic computational graph, user friendly and emphasizing on research and development. PyTorch’s key features include:
- Dynamic computational graphs: Another important point for discussion is that the computational graph in PyTorch can be rewired during the construction of the model, or during the experiment.
- Pythonic interface: One of the report’s main findings was that PyTorch supports developers who are familiar with Python by using a syntax that is similar to Python code.
- Strong research focus: PyTorch is currently under the active contribution from the research community hence the most favored deep learning frameworks for research.
- GPU acceleration: As other deep learning frameworks, PyTorch also uses the GPU contribution successfully leading to the work for enhancing the efficiency and speed of the model training.
Keras
Keras is an open source neural networks library written in python which runs on top of other low level neural networks API’s. It also offers a clean and natural style for creating and training model based on deep learning. Keras’s key features include:
- Simplicity and ease of use: Due to the offered abstraction, the Keras can be used by people who do not have a great experience in deep learning and machine learning in general.
- Modularity and extensibility: However, Keras enables you build the models out of smaller blocks and in that way a user is able to try out different architectures of the models.
- Runs on top of TensorFlow, Theano, or CNTK: Today, Keras supports several backend engines, and they are TensorFlow, Theano and Microsoft’s Cognitive Toolkit (CNTK).
Other Notable Frameworks
In addition to TensorFlow, PyTorch, and Keras, several other deep learning frameworks deserve mention:
- Caffe: A convolutional neural network structure that was designed by the Berkeley Vision and Learning Center (BVLC), and is best utilized for image categorization.
- Theano: An open source programming utility package in the Python language through which it is possible to describe, improve, and appraise mathematical formulas with multi-dimensional arrays.
- MXNet: An adaptable and optimized deep learning environment for use with different languages and expandable across different platforms.
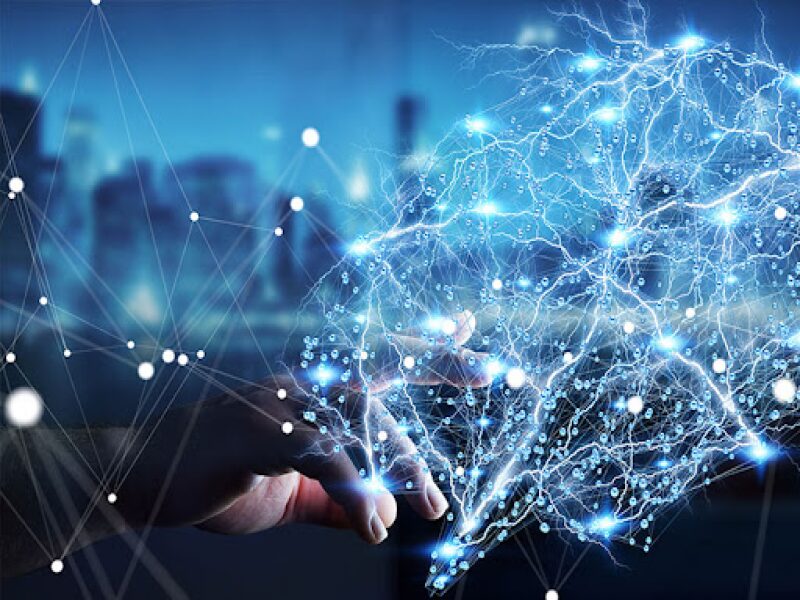
Applications of Deep Learning for Cloud Platforms
Google or Microsoft cloud services are well suited for deep learning because they don’t take specialized and costly equipment or IT personnel.
- Google Colab: A web service where you can access GPUs and TPUs for free, which allow its user to run and test deep learning models online.
- Amazon Sage Maker: A full service and self-service offering, which includes tools and flows for building, training and deploying ML models including Deep learning at scale.
- Microsoft Azure: The open-source hardware platform to implement machine learning can be categorized as the following:- Deep learning frameworks- Pre-trained models- Model-Deployment & Management- etc.
Many of these frameworks, tools, and cloud platforms have made it easier and affordable for anyone to use deep learning and be creative with it.
In the next part of this paper, we will discuss the problem with deep learning and the future developments of deep learning.
Challenges and Future of Deep Learning
Despite the effectiveness of deep learning, many problems can be encountered when developing it. This is in terms of data demands, computational capacity and issues of explain-ability, ethics, and bias. Here we would like to provide an overview of some of the major issues with deep learning and discuss the future developments.
Data Requirements
Deep learning models are a kind of models which demand large amount of data. However, the general disadvantage of the supervised learning methods is to harness of big amounts of labeled data. Such datasets are often difficult to obtain and preprocess, which may take much time and can be costly. As already pointed out, privacy concerns and regulatory compliance make it challenging to obtain sufficiently many labelled data points even when there are plenty of data from the respective domain, such as with healthcare data or data from autonomous vehicles.
Computational Resources
As mentioned before, tuning complex deep learning models can be computationally very greedy, needing high end GPUs, if not also other specialized chips. This can indeed be a problem to those individuals and firms who have small financial means. Nevertheless, with the emergence of cloud computing and initialization of superior algorithms this issue is not witnessing a big problem anymore.
This allows for endeavoring for explain ability and interpretability.
The decision-making process is opaque, and the architectures themselves are often called ‘black boxes’. Especially, the factors such as unexplainably and interpretability can be considered as an issue at least in the extent that they are linked to quite important spheres of the healthcare or the financial sector. There has been remarkable research focusing on building methodologies for explaining the behaviors of deep learning models.
Ethical Considerations
But as deep learning finds its place in our daily activities we are faced with critical ethical issues. 7 G: Huxtable on bias in algorithms, possible wrongful use of the above AI technologies and the ramifications arising from the automation of employment service. This means that deep learning models should be used correctly, and basically they must be defined as fair and accountable.
Deep Learning future trends
Hence there are continuous development of the topic in the field of deep learning owing to the ever-growing research and innovation. Here are some of the future trends that are shaping the field:
- Transfer Learning: This technique was developed using pre-trained models on extensive data and then training them further on slightly restricted, special data sets. Indeed the use of transfer learning can significantly cut down on the amount of data and amount of computation that is required to train deep learning models that in turn makes the models more manageable to train.
- Reinforcement Learning: This approach entails agents that learn when and how to act in a specific environment by providing a reinforcer once a targeted type of behavior is displayed and withholding the reinforcer or providing a negative reinforcer for undesired behavior is exhibited. Reinforcement learning has yielded good performances in areas such as game playing, robotics and self autonomous systems.
- Generative Adversarial Networks (GANs): of the generator and the discriminator that operate in a contrasting manner. The generator generates fake data and the discriminator attempts to label fake data and actual data as fake. GANs are believed to have shown impressive performance for producing Did Appearance, images, videos, and even music.
- Explainable AI (XAI): XAI is the type of AI technique that is focused to increase the interpretability of deep learning, while it offers explanations to customers about a model’s choices it has made. This is important more so in building the much needed trust in artificial intelligence powered systems and the proper implementation of such systems.
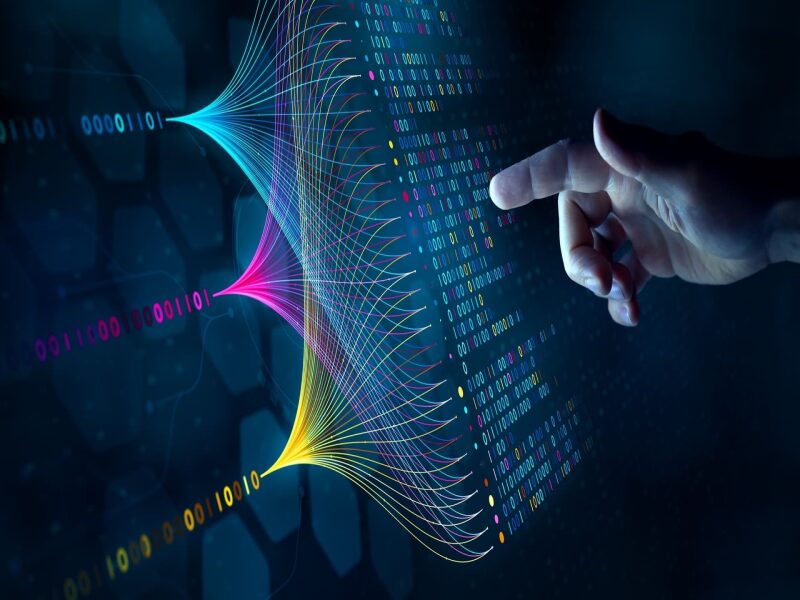
Conclusion
Author of this piece opines that application of deep learning with the help of models based on neural networks brought a tectonic shift worldwide in different fields. The potential to process intricate data, detect important features, and still get superior results has made deep learning an impressive tool in image recognition, natural language interactions, medical technologies, and self-driving automobiles, alongside many other fields. Of course, today deep learning still has several drawbacks; however, the future looks really encouraging: there are many promising developments and trends on the horizon.
While proceeding to the deep learning, we need to discuss the potential social impacts that might occur due to this advancement and work towards the better future development and using of AI technologies. Deep learning can be used, as in the dreams of human authors, to create an AI future capable of improving people’s lives while remaining ethical.
Call to Action
To this end, we recommend that interested readers explore the deep world of Deep Learning even further. Use added resources available in the internet for more information and enrollment to courses and tutorials. It is wise to be charmed as well as keep inquisitive while deploying other deep learning frameworks, tools, and develop them to escalate the growth of this revolutionary field across the world.
Just imagine that profound learning potential is not only based on the technological advancement but also on the usage of such technologies properly and ethically. This technological advance means that we can help to forge an AI-literate future, which will use this powerful technology as the key to positive change in the near and distant future.